Open Access
ARTICLE
Statistical Time Series Forecasting Models for Pandemic Prediction
1 Department of Electrical Engineering, Faculty of Engineering, Ahram Canadian University, 6th October City, Giza, Egypt
2 Security Engineering Lab, Computer Science Department, Prince Sultan University, Riyadh, 11586, Saudi Arabia
3 Department of Electronics and Electrical Communications Engineering, Faculty of Electronic Engineering, Menoufia University, Menouf, 32952, Egypt
4 Department of Information Technology, College of Computer and Information Sciences, Princess Nourah bint Abdulrahman University, P.O. Box 84428, Riyadh, 11671, Saudi Arabia
5 Electronics and Communications Engineering Department, College of Engineering and Technology, Arab Academy for Science, Technology and Maritime Transport, Alexandria, 1029, Egypt
* Corresponding Author: Abeer D. Algarni. Email:
Computer Systems Science and Engineering 2023, 47(1), 349-374. https://doi.org/10.32604/csse.2023.037408
Received 02 November 2022; Accepted 17 February 2023; Issue published 26 May 2023
Abstract
COVID-19 has significantly impacted the growth prediction of a pandemic, and it is critical in determining how to battle and track the disease progression. In this case, COVID-19 data is a time-series dataset that can be projected using different methodologies. Thus, this work aims to gauge the spread of the outbreak severity over time. Furthermore, data analytics and Machine Learning (ML) techniques are employed to gain a broader understanding of virus infections. We have simulated, adjusted, and fitted several statistical time-series forecasting models, linear ML models, and nonlinear ML models. Examples of these models are Logistic Regression, Lasso, Ridge, ElasticNet, Huber Regressor, Lasso Lars, Passive Aggressive Regressor, K-Neighbors Regressor, Decision Tree Regressor, Extra Trees Regressor, Support Vector Regressions (SVR), AdaBoost Regressor, Random Forest Regressor, Bagging Regressor , AuoRegression, MovingAverage, Gradient Boosting Regressor, Autoregressive Moving Average (ARMA), Auto-Regressive Integrated Moving Averages (ARIMA), SimpleExpSmoothing, Exponential Smoothing, Holt-Winters, Simple Moving Average, Weighted Moving Average, Croston, and naive Bayes. Furthermore, our suggested methodology includes the development and evaluation of ensemble models built on top of the best-performing statistical and ML-based prediction methods. A third stage in the proposed system is to examine three different implementations to determine which model delivers the best performance. Then, this best method is used for future forecasts, and consequently, we can collect the most accurate and dependable predictions.Keywords
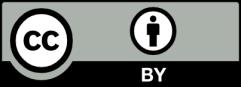