Open Access
ARTICLE
Deep Autoencoder-Based Hybrid Network for Building Energy Consumption Forecasting
1 Department of Software Convergence, Sejong University, Seoul, 143-747, South Korea
2 Department of Convergence Engineering for Intelligent Drone, Sejong University, Seoul, 143-747, South Korea
* Corresponding Author: Sung Wook Baik. Email:
(This article belongs to the Special Issue: Metaheuristics Optimization for Real-World Applications)
Computer Systems Science and Engineering 2024, 48(1), 153-173. https://doi.org/10.32604/csse.2023.039407
Received 27 January 2023; Accepted 11 April 2023; Issue published 26 January 2024
Abstract
Energy management systems for residential and commercial buildings must use an appropriate and efficient model to predict energy consumption accurately. To deal with the challenges in power management, the short-term Power Consumption (PC) prediction for household appliances plays a vital role in improving domestic and commercial energy efficiency. Big data applications and analytics have shown that data-driven load forecasting approaches can forecast PC in commercial and residential sectors and recognize patterns of electric usage in complex conditions. However, traditional Machine Learning (ML) algorithms and their features engineering procedure emphasize the practice of inefficient and ineffective techniques resulting in poor generalization. Additionally, different appliances in a home behave contrarily under distinct circumstances, making PC forecasting more challenging. To address these challenges, in this paper a hybrid architecture using an unsupervised learning strategy is investigated. The architecture integrates a one-dimensional Convolutional Neural Network (CNN) based Autoencoder (AE) and online sequential Extreme Learning Machine (ELM) for commercial and residential short-term PC forecasting. First, the load data of different buildings are collected and cleaned from various abnormalities. A subsequent step involves AE for learning a compressed representation of spatial features and sending them to the online sequential ELM to learn nonlinear relations and forecast the final load. Finally, the proposed network is demonstrated to achieve State-of-the-Art (SOTA) error metrics based on two benchmark PC datasets for residential and commercial buildings. The Mean Square Error (MSE) values obtained by the proposed method are 0.0147 and 0.0121 for residential and commercial buildings datasets, respectively. The obtained results prove that our model is suitable for the PC prediction of different types of buildings.Keywords
Cite This Article
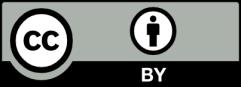