Open Access
ARTICLE
TEAM: Transformer Encoder Attention Module for Video Classification
1 Department of Artificial Intelligence, Hanyang University, Seoul, Korea
2 Department of Computer Science, Hanyang University, Seoul, Korea
* Corresponding Author: Yong Suk Choi. Email:
Computer Systems Science and Engineering 2024, 48(2), 451-477. https://doi.org/10.32604/csse.2023.043245
Received 26 June 2023; Accepted 26 October 2023; Issue published 19 March 2024
Abstract
Much like humans focus solely on object movement to understand actions, directing a deep learning model’s attention to the core contexts within videos is crucial for improving video comprehension. In the recent study, Video Masked Auto-Encoder (VideoMAE) employs a pre-training approach with a high ratio of tube masking and reconstruction, effectively mitigating spatial bias due to temporal redundancy in full video frames. This steers the model’s focus toward detailed temporal contexts. However, as the VideoMAE still relies on full video frames during the action recognition stage, it may exhibit a progressive shift in attention towards spatial contexts, deteriorating its ability to capture the main spatio-temporal contexts. To address this issue, we propose an attention-directing module named Transformer Encoder Attention Module (TEAM). This proposed module effectively directs the model’s attention to the core characteristics within each video, inherently mitigating spatial bias. The TEAM first figures out the core features among the overall extracted features from each video. After that, it discerns the specific parts of the video where those features are located, encouraging the model to focus more on these informative parts. Consequently, during the action recognition stage, the proposed TEAM effectively shifts the VideoMAE’s attention from spatial contexts towards the core spatio-temporal contexts. This attention-shift manner alleviates the spatial bias in the model and simultaneously enhances its ability to capture precise video contexts. We conduct extensive experiments to explore the optimal configuration that enables the TEAM to fulfill its intended design purpose and facilitates its seamless integration with the VideoMAE framework. The integrated model, i.e., VideoMAE + TEAM, outperforms the existing VideoMAE by a significant margin on Something-Something-V2 (71.3% vs. 70.3%). Moreover, the qualitative comparisons demonstrate that the TEAM encourages the model to disregard insignificant features and focus more on the essential video features, capturing more detailed spatio-temporal contexts within the video.Keywords
Cite This Article
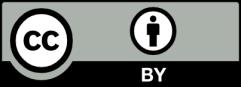