Open Access
ARTICLE
Tomato Leaf Disease Identification and Detection Based on Deep Convolutional Neural Network
1 Department of Electronics and Information Engineering, Tongji University, Shanghai, 201804, China
2 BEACON Center for the study of Evolution in Action, Michigan State University, East Lansing, MI 48824, USA
* Corresponding Author: Lihong Xu. Email:
Intelligent Automation & Soft Computing 2021, 28(2), 561-576. https://doi.org/10.32604/iasc.2021.016415
Received 01 January 2021; Accepted 26 February 2021; Issue published 01 April 2021
Abstract
Deep convolutional neural network (DCNN) requires a lot of data for training, but there has always been data vacuum in agriculture, making it difficult to label all existing data accurately. Therefore, a lightweight tomato leaf disease identification network supported by Variational auto-Encoder (VAE) is proposed to improve the accuracy of crop leaf disease identification. In the lightweight network, multi-scale convolution can expand the network width, enrich the extracted features, and reduce model parameters such as deep separable convolution. VAE makes full use of a large amount of unlabeled data to achieve unsupervised learning, and then uses labeled data for supervised disease identification. However, in the actual model deployment and production environment, VAE doesn’t require additional calculation and storage consumption, because it is not used in the calculation of the application phase. Compared with the classification network that only uses labeled data, the generalization effect and identification accuracy of this proposed method are enhanced. Especially in the case of fewer labeled samples, the identification accuracy has increased from 56.13% to 78.03%, and in the case of many labeled samples, the identification accuracy also shows a rise. We have fully confirmed the effectiveness of the lightweight network and VAE enhancement strategy: the correct detection rate of disease category by this method is 94.17%, and only 0.42% of the diseased leaves are misidentified as healthy leaves; the correct detection rate of healthy leaves is 98.27%, and only 1.73% of healthy leaves are misidentified as diseased leaves.Keywords
Cite This Article
Citations
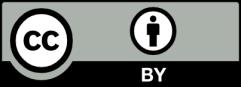