Open Access
ARTICLE
Combining CNN and Grad-Cam for COVID-19 Disease Prediction and Visual Explanation
1 SSDIA Laboratory, ENSET of Mohammedia, Hassan II University of Casablanca, Mohammedia, 28820, Morocco
2 STIE Team, CRMEF Casablanca-Settat, Provincial Section of El Jadida, El Jadida, 24000, Morocco
3 College of Computer Science and Engineering, Taibah University, Medina, 344, Saudi Araibia
4 Department of Information Systems, College of Computer Engineering and Sciences, Prince Sattam bin Abdulaziz University, Al-Kharj, 11942, Saudi Arabia
* Corresponding Author: Bouchaib Cherradi. Email:
(This article belongs to the Special Issue: New Trends in Artificial Intelligence and Deep learning for Instrumentation, Sensors, and Robotics)
Intelligent Automation & Soft Computing 2022, 32(2), 723-745. https://doi.org/10.32604/iasc.2022.022179
Received 30 July 2021; Accepted 02 September 2021; Issue published 17 November 2021
Abstract
With daily increasing of suspected COVID-19 cases, the likelihood of the virus mutation increases also causing the appearance of virulent variants having a high level of replication. Automatic diagnosis methods of COVID-19 disease are very important in the medical community. An automatic diagnosis could be performed using machine and deep learning techniques to analyze and classify different lung X-ray images. Many research studies proposed automatic methods for detecting and predicting COVID-19 patients based on their clinical data. In the leak of valid X-ray images for patients with COVID-19 datasets, several researchers proposed to use augmentation techniques to bypass this limitation. However, the obtained results by augmentation techniques are not efficient to be projected for the real world. In this paper, we propose a convolutional neural network (CNN)-based method to analyze and distinguish COVID-19 cases from other pneumonia and normal cases using the transfer learning technique. To help doctors easily interpret the results, a recent visual explanation method called Gradient-weighted Class Activation Mapping (Grad-CAM) is applied for each class. This technique is used in order to highlight the regions of interest on the X-ray image, so that, the model prediction result can be easily interpreted by the doctors. This method allows doctors to focus only on the important parts of the image and evaluate the efficiency of the concerned model. Three selected deep learning models namely VGG16, VGG19, and MobileNet, were used in the experiments with transfer learning technique. To bypass the limitation of the leak of lung X-ray images of patients with COVID-19 disease, we propose to combine several different datasets in order to assemble a new dataset with sufficient real data to accomplish accurately the training step. The best results were obtained using the tuned VGG19 model with 96.97% accuracy, 100% precision, 100% F1-score, and 99% recall.Keywords
Cite This Article
Citations
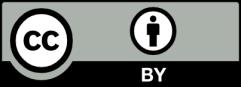