Open Access
ARTICLE
Performance Comparison of Deep and Machine Learning Approaches Toward COVID-19 Detection
1 Department of Software Engineering, Istanbul Sabahattin Zaim University, Istanbul, 34303, Turkey
2 Department of Software Engineering, Nisantasi University, Istanbul, 34398, Turkey
3 Department of Computer Science, College of Computer Engineering and Sciences, Prince Sattam bin Abdulaziz University, Al-Kharj, 11942, Saudi Arabia
4 Department of Electrical and Computer Engineering, New York University (NYU), Abu Dhabi, 129188, United Arab Emirates
5 College of Computer Engineering and Sciences, Prince Sattam bin Abdulaziz University, Al-Kharj, 11942, Saudi Arabia
6 Department of Computer Engineering, Istanbul Topkapi University, Istanbul, 34087, Turkey
7 Baqai Institute of Hematology, Baqai Medical University, Karachi, 75340, Pakistan
* Corresponding Author: Jawad Rasheed. Email:
(This article belongs to the Special Issue: Artificial Intelligence based Healthcare Systems)
Intelligent Automation & Soft Computing 2023, 37(2), 2247-2261. https://doi.org/10.32604/iasc.2023.036840
Received 13 October 2022; Accepted 24 February 2023; Issue published 21 June 2023
Abstract
The coronavirus (COVID-19) is a disease declared a global pandemic that threatens the whole world. Since then, research has accelerated and varied to find practical solutions for the early detection and correct identification of this disease. Several researchers have focused on using the potential of Artificial Intelligence (AI) techniques in disease diagnosis to diagnose and detect the coronavirus. This paper developed deep learning (DL) and machine learning (ML) -based models using laboratory findings to diagnose COVID-19. Six different methods are used in this study: K-nearest neighbor (KNN), Decision Tree (DT) and Naive Bayes (NB) as a machine learning method, and Deep Neural Network (DNN), Convolutional Neural Network (CNN), and Long-term memory (LSTM) as DL methods. These approaches are evaluated using a dataset obtained from the Israelita Albert Einstein Hospital in Sao Paulo, Brazil. This data consists of 5644 laboratory results from different patients, with 10% being Covid-19 positive cases. The dataset includes 18 attributes that characterize COVID-19. We used accuracy, f1-score, recall and precision to evaluate the different developed systems. The obtained results confirmed these approaches’ effectiveness in identifying COVID-19, However, ML-based classifiers couldn't perform up to the standards achieved by DL-based models. Among all, NB performed worst by hardly achieving accuracy above 76%, Whereas KNN and DT compete by securing 84.56% and 85% accuracies, respectively. Besides these, DL models attained better performance as CNN, DNN and LSTM secured more than 90% accuracies. The LTSM outperformed all by achieving an accuracy of 96.78% and an F1-score of 96.58%.Keywords
Cite This Article
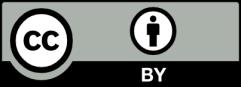