Open Access
ARTICLE
Explainable AI and Interpretable Model for Insurance Premium Prediction
Department of Computer and Information Science, University of Strathclyde, Glasgow, UK
* Corresponding Authors: Umar Abdulkadir Isa. Email: ; Anil Fernando. Email:
Journal on Artificial Intelligence 2023, 5, 31-42. https://doi.org/10.32604/jai.2023.040213
Received 09 March 2023; Accepted 23 May 2023; Issue published 11 August 2023
Abstract
Traditional machine learning metrics (TMLMs) are quite useful for the current research work precision, recall, accuracy, MSE and RMSE. Not enough for a practitioner to be confident about the performance and dependability of innovative interpretable model 85%–92%. We included in the prediction process, machine learning models (MLMs) with greater than 99% accuracy with a sensitivity of 95%–98% and specifically in the database. We need to explain the model to domain specialists through the MLMs. Human-understandable explanations in addition to ML professionals must establish trust in the prediction of our model. This is achieved by creating a model-independent, locally accurate explanation set that makes it better than the primary model. As we know that human interaction with machine learning systems on this model’s interpretability is more crucial. For supporting set validations in model selection insurance premium prediction. In this study, we proposed the use of the (LIME and SHAP) approach to understand research properly and explain a model developed using random forest regression to predict insurance premiums. The SHAP algorithm’s drawback, as seen in our experiments, is its lengthy computing time—to produce the findings, it must compute every possible combination. In addition, the experiments conducted were intended to focus on the model’s interpretability and explain its ability using LIME and SHAP, not the insurance premium charge prediction. Three experiments were conducted through experiment, one was to interpret the random forest regression model using LIME techniques. In experiment 2, we used the SHAP technique to interpret the model insurance premium prediction (IPP).Keywords
Cite This Article
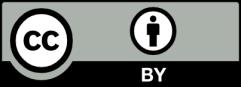