Open Access
ARTICLE
An Improved Deep Structure for Accurately Brain Tumor Recognition
1 Department of Communications and Electronics Engineering, MISR Higher Institute for Engineering and Technology, Mansoura, 35516, Egypt
2 Department of Electronics and Communications, Delta Higher Institute for Engineering and Technology, Mansoura, 35516, Egypt
3 Department of Computer Sciences, College of Computer and Information Sciences, Princess Nourah bint Abdulrahman University, P.O. Box 84428, Riyadh, 11671, Saudi Arabia
* Corresponding Author: Faten Khalid Karim. Email:
Computer Systems Science and Engineering 2023, 46(2), 1597-1616. https://doi.org/10.32604/csse.2023.034375
Received 15 July 2022; Accepted 23 November 2022; Issue published 09 February 2023
Abstract
Brain neoplasms are recognized with a biopsy, which is not commonly done before decisive brain surgery. By using Convolutional Neural Networks (CNNs) and textural features, the process of diagnosing brain tumors by radiologists would be a noninvasive procedure. This paper proposes a features fusion model that can distinguish between no tumor and brain tumor types via a novel deep learning structure. The proposed model extracts Gray Level Co-occurrence Matrix (GLCM) textural features from MRI brain tumor images. Moreover, a deep neural network (DNN) model has been proposed to select the most salient features from the GLCM. Moreover, it manipulates the extraction of the additional high levels of salient features from a proposed CNN model. Finally, a fusion process has been utilized between these two types of features to form the input layer of additional proposed DNN model which is responsible for the recognition process. Two common datasets have been applied and tested, Br35H and FigShare datasets. The first dataset contains binary labels, while the second one splits the brain tumor into four classes; glioma, meningioma, pituitary, and no cancer. Moreover, several performance metrics have been evaluated from both datasets, including, accuracy, sensitivity, specificity, F-score, and training time. Experimental results show that the proposed methodology has achieved superior performance compared with the current state of art studies. The proposed system has achieved about 98.22% accuracy value in the case of the Br35H dataset however, an accuracy of 98.01% has been achieved in the case of the FigShare dataset.Keywords
Cite This Article
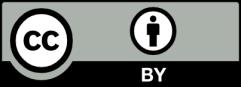