Open Access
ARTICLE
Classification of Multi-view Digital Mammogram Images Using SMO-WkNN
1 Department of Computer Science and Engineering, Saveetha School of Engineering, Saveetha Institute of Medical and Technical Sciences (Deemed to be University), Chennai, Tamilnadu, India
2 Department of Information Technology, Saveetha School of Engineering, Saveetha Institute of Medical and Technical Sciences (Deemed to be University), Chennai, Tamilnadu, India
* Corresponding Author: P. Malathi. Email:
Computer Systems Science and Engineering 2023, 46(2), 1741-1758. https://doi.org/10.32604/csse.2023.035185
Received 10 August 2022; Accepted 15 November 2022; Issue published 09 February 2023
Abstract
Breast cancer (BCa) is a leading cause of death in the female population across the globe. Approximately 2.3 million new BCa cases are recorded globally in females, overtaking lung cancer as the most prevalent form of cancer to be diagnosed. However, the mortality rates for cervical and BCa are significantly higher in developing nations than in developed countries. Early diagnosis is the only option to minimize the risks of BCa. Deep learning (DL)-based models have performed well in image processing in recent years, particularly convolutional neural network (CNN). Hence, this research proposes a DL-based CNN model to diagnose BCa from digitized mammogram images. The main objective of this research is to develop an accurate and efficient early diagnosis model for BCa detection. This proposed model is a multi-view-based computer-aided diagnosis (CAD) model, which performs the diagnosis of BCa on multi-views of mammogram images like medio-lateral-oblique (MLO) and cranio-caudal (CC). The digital mammogram images are collected from the digital database for screening mammography (DDSM) dataset. In preprocessing, median filter and contrast limited adaptive histogram equalization (CLAHE) techniques are utilized for image enhancement. After preprocessing, the segmentation is performed using the region growing (RG) algorithm. The feature extraction is carried out from the segmented images using a pyramidal histogram of oriented gradients (PHOG) and the AlextNet model. Finally, the classification is performed using the weighted k-nearest neighbor (WkNN) optimized with sequential minimal optimization (SMO). The classified images are evaluated based on accuracy, recall, precision, specificity, f1-score, and mathews correlation coefficient (MCC). Additionally, the false positive and error rates are evaluated. The proposed model obtained 98.57% accuracy, 98.61% recall, 99.25% specificity, 98.63% precision, 97.93% f1-score, 96.26% MCC, 0.0143 error rate, and 0.0075 false positive rate (FPR). Compared to the existing models, the research model has obtained better performances and outperformed the other models.Keywords
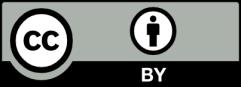