Open Access
ARTICLE
Detection of Alzheimer’s Disease Progression Using Integrated Deep Learning Approaches
1 Department of Information and Communication Technology, Manipal Institute of Technology, Manipal Academy of Higher Education, Manipal, 576104, India
2 Department of Swasthavritta and Yoga, J S Ayurved Mahavidyalay, Nadiad, 387001, India
* Corresponding Author: Nisha P. Shetty. Email:
(This article belongs to the Special Issue: Artificial Intelligence based Healthcare Systems)
Intelligent Automation & Soft Computing 2023, 37(2), 1345-1362. https://doi.org/10.32604/iasc.2023.039206
Received 14 January 2023; Accepted 28 February 2023; Issue published 21 June 2023
Abstract
Alzheimer’s disease (AD) is an intensifying disorder that causes brain cells to degenerate early and destruct. Mild cognitive impairment (MCI) is one of the early signs of AD that interferes with people’s regular functioning and daily activities. The proposed work includes a deep learning approach with a multimodal recurrent neural network (RNN) to predict whether MCI leads to Alzheimer’s or not. The gated recurrent unit (GRU) RNN classifier is trained using individual and correlated features. Feature vectors are concatenated based on their correlation strength to improve prediction results. The feature vectors generated are given as the input to multiple different classifiers, whose decision function is used to predict the final output, which determines whether MCI progresses onto AD or not. Our findings demonstrated that, compared to individual modalities, which provided an average accuracy of 75%, our prediction model for MCI conversion to AD yielded an improvement in accuracy up to 96% when used with multiple concatenated modalities. Comparing the accuracy of different decision functions, such as Support Vector Machine (SVM), Decision tree, Random Forest, and Ensemble techniques, it was found that that the Ensemble approach provided the highest accuracy (96%) and Decision tree provided the lowest accuracy (86%).Keywords
Cite This Article
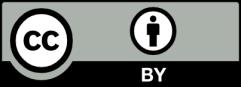