Open Access
ARTICLE
Restructuring Tilth Layers Can Change the Microbial Community Structure and Affect the Occurrence of Verticillium Wilt in Cotton Field
Institute of Cotton, Key Laboratory of Biology and Genetic Improvement of Cotton in Huanghuaihai Semiarid Area, Ministry of Agriculture, National Cotton Improvement Center Hebei Branch, Shijiazhuang, 050051, China
* Corresponding Authors: Yongqiang Wang. Email: ; Hong Qi. Email:
Phyton-International Journal of Experimental Botany 2023, 92(10), 2841-2860. https://doi.org/10.32604/phyton.2023.030465
Received 07 April 2023; Accepted 03 July 2023; Issue published 15 September 2023
Abstract
Restructuring tilth layers (RTL) is a tillage method that exchanges the 0–20 and 20–40 cm soil layers that can be applied during cotton cultivation to increase cotton yield, eliminate weeds and alleviate severe disease, including Verticillium wilt. However, the mechanism by which RTL inhibits Verticillium wilt is unclear. Therefore, we investigated the distribution of microbial communities after rotary tillage (CK) and RTL treatments to identify the reasons for the reduction of Verticillium wilt in cotton fields subjected to RTL. Illumina high-throughput sequencing was used to sequence the bacterial and fungal genes. The disease incidence and severity of Verticillium wilt decreased by 28.57% and 42.64%, respectively, after RTL. Moreover, RTL significantly enhanced bacterial richness and evenness at 20–40 cm and -reduced the differences in fungal evenness and richness between soil depths of 0–20 and 20–40 cm. The number of Verticillium dahliae decreased, while the relative abundance of biocontrol bacteria such as Bacillus and Pseudoxanthomonas increased significantly following RTL. Overall RTL improved bacterial diversity, decreased the number of Verticillium dahliae and increased the relative abundance of biocontrol bacteria, which may have suppressed the occurrence of Verticillium wilt in cotton fields.Keywords
Supplementary Material
Supplementary Material FileTillage, which is one of the main components of traditional farming, dates back thousands of years [1]. Tillage plays a vital role in agricultural systems by providing suitable soil structure for seeds, repressing pests and weeds [2] and influencing crop root growth and yield [3]. Tillage not only improves the physical and chemical properties of soil, but also changes soil microbial communities [4,5]. Moreover, different tillage practices can promote the growth of microbes with different niches and alter the vertical distribution of soil microbial communities [6,7].
Rotary tillage is an influential management practice that improves soil structure [8] and has therefore long been applied in the Huang-Huai-Hai cotton region of China [9]. The tillage depth of rotary tillage is 15 cm in general. However, continuous long-term rotary tillage results in a hard ploughing pan and increased subsoil compaction, which restricts cotton root penetration, increases soil surface water evaporation, and aggravates disease occurrence [10–12]. In recent years, a new tillage practice, restructuring tilth layers (RTL), has been implemented in cotton cultivation in China. This practice completely exchanges the topsoil (0–20 cm) with the subsoil (20–40 cm) vertically and disturbs the soil below 40 cm. Notably, deep tillage is conducted to mix the soil in tilth layer, while RTL is conducted to exchange the two soil layers (Fig. S1). Previous research has shown that RTL could increase cotton yield [13], improve soil water conservation in deep soil (below 20 cm) [14], and eliminate weeds [15]. Moreover, RTL has been shown to effectively alleviate severe diseases, especially Verticillium wilt [16]. However, the mechanisms responsible for the soil microbial of RTL effects remain unclear.
The pathogen responsible for Verticillium wilt of cotton in China is Verticillium dahlia Kleb [17], which produces a large number of microsclerotia in infected plant tissue. As crop residues decompose, these microsclerotia are released into the soil, where they can survive for 10 years or more [18,19]. The severity of Verticillium wilt disease depends largely on the number of V. dahliae in soil [20,21]. For example, a higher concentration of V. dahliae in soil resulted in severer in strawberry [20]. The development of Verticillium wilt is also influenced by various environmental factors such as soil moisture and microbial community composition [22,23]. Infection with V. dahliae and subsequent disease development can lead to large changes in cotton microbiomes [24], while beneficial microbes can inhibit soil pathogens and reduce the incidence of soil borne plant diseases [25].
In this study, we conducted a field experiment to explore the effects of RTL on the occurrence of Verticillium wilt in cotton field and applied high-throughput sequencing to investigate the mechanisms of this effects. We hypothesized that 1) the occurrence of Verticillium wilt would decrease after RTL treatment; 2) such decreases would be associated with changes in the microbial community related to Verticillium wilt after RTL treatment; 3) decrease would be related to the changes in the V. dahliae population after RTL treatments. The results of this study can provide further insight into the spatial and temporal variations of soil microorganisms during RTL.
This study was conducted in 2020 during cotton growing season at the Weixian Agricultural Experimental Station of the Institute of Cotton, Hebei Academy and Forest Science in Hebei Province, China (36°98′N, 115°25′E). The area is characterized by a warm and mild temperate continental semi-arid monsoon climate, seasonal changes, and sufficient sunshine. A continuous rotary tillage cotton field underlaid by sandy loam with base nutrients of 11.3 g kg−1 organic matter, 0.9 g kg−1 total nitrogen, 45.6 mg kg−1 available phosphorus and 45.6 mg kg−1 available potassium in the 0–20 cm layer, that was naturally infested with V. dahliae, was selected as the experimental site.
“Hengmian HD008”, a susceptible cultivar, was selected as the experimental material. The seeding date was April 25, 2020 and the harvest date was September 15, 2020. The planting depth was 5 cm, the planting density was 66,000 plants/hm2, and the row spacing was 76 cm. The fertilizer regime consisted of application of N fertilizer at 180 kg/hm2, P2O5 at 90 kg/hm2, K2O at 120 kg/hm2. Weeds control and irrigation management were conducted according to local production techniques.
The experiment consisted of two treatments. Rotary tillage with a tillage depth of 15 cm was the control (CK), while RTL using rotary deep plowing to exchange the 0–20 cm soil layer with the 20–40 cm soil layer and loosen the 40–55 cm soil layer was the treatment. Three 8 m × 6 m plots were established in each treatment and a randomized block approach was employed.
The rotary deep plow was equipped with two sets of plowshares, that were symmetrically distributed. Each group of plowshares consisted of one main plowshare and one auxiliary plowshare, with a deep loosening shovel on the main plowshare. The main plowshare was in front of the auxiliary plowshare (Fig. S2). During operation, the rotary deep plow was pulled by a tractor. The main plowshare was responsible for trenching to a depth of 40 cm, while the deep loosening shovel loosened the soil at 40–55 cm. The auxiliary plowshare turned the 0–20 cm soil layer next to the ditch into the furrow. When the auxiliary plowshare reached the boundary of the community, the tractor turned around and flipped the 20–40 cm soil layer into the furrow, achieving the replacement of the 0–20 cm soil layer with 20–40 cm soil layer and loosening of the 40–55 cm soil layer.
The five-spot-sampling method [26] was used to collect samples from all plots on June 20 (budding stage) and August 21 (boll opening stage), 2020. Twelve soils samples were collected from 0–20 and 20–40 cm in June (three replicates in one plot, 2 × 2 × 3 = 12) and 12 soil samples were collected from 0–20 and 20–40 cm in August (three replicates per plot, 2 × 2 × 3 = 12). All 24 soil samples were collected using an auger (5 cm diameter and 20 cm long). Plant residues, stones, and other impurities were removed from soil samples, then fully mixed to form composite soil samples. Samples were divided into two parts, one that was sieved through 2-mm mesh sieves for physical analysis and another that was stored at −80°C until DNA extraction. Another 24 soil samples were collected using a ring knife on June 20 (budding stage) and August 21 (boll opening stage), 2020. Twelve soil samples were collected from 0–20 and 20–40 cm in June (3 replicates in one plot, 2 × 2 × 3 = 12) and Twelve soil samples were collected from 0–20 and 20–40 cm in August (three replicates per plot, 2 × 2 × 3 = 12). Following collection, samples were stored intact until they were returned to the laboratory and analyzed for soil bulk density, capillary porosity, and non-capillary porosity.
2.4 Measurement of Soil Physical Properties
The bulk density of soil from the 0–20 and 20–40 cm soil layers was measured using the cutting ring method [27]. Briefly, samples were placed into an aluminum box and then dried in an oven (105°C) to constant weight, after which the soil bulk density was calculated. The formula for calculating the soil bulk density was as follows:
where SBD is the soil bulk density, Mt is the weight of the aluminum box and wet soil sample, Ma is the weight of the aluminum box, Vr is the volume of the ring knife, Mw is the weight of the fresh soil sample, and Md is the weight of the dried soil sample.
The specific gravity of soil was determined by the pycnometer method [28]. Briefly, soil samples were placed into water to remove the air, after which the volume of liquid that replaced the air in the soil was calculated. The dry soil weight (105°C) was then divided by the volume to obtain the specific gravity of the soil. The formula used to calculate the specific gravity of the soil was as follows:
where SGS was the specific gravity of soil, Ms was the weight of the dried soil sample, St was the specific gravity of water at t°C, Ma was the weight of the pycnometer and water at t°C, and Mta was the weight of the pycnometer, water, and soil sample at t°C.
Next, the soil porosity was calculated from the soil bulk density and soil specific gravity as follows:
where TSP was the total soil porosity.
2.4.3 Capillary Porosity and Non-Capillary Porosity
Capillary porosity was also calculated by the cutting ring method [29]. Briefly, undisturbed soil samples were collected using a ring knife and then placed in a tray with a water depth of 2–3 mm for 12 h, after which each soil sample was weighed. Next, the sample was dried in an oven (105°C) to constant weight, after which the mass of the dried soil sample was calculated. The formulas used to calculate the soil capillary porosity and non-capillary porosity were as follows:
where CP was the capillary porosity, Mw was the weight of soil samples after water absorption and the weight of the ring knife, Md was the weight of the dried soil sample, Mr was the weight of the ring knife, Vr was the volume of the ring knife, and NCP was the non-capillary porosity.
Soil temperature was recorded on June 20 (bud stage) and August 21 (boll opening stage), 2020 using a geothermometer set at soil depths of 10 and 30 cm to represent the temperature of topsoil and subsoil, respectively.
The soil moisture content on April 16 (pre-planting), May 22 (seeding stage), June 20 (bud stage), July 21 (flower and boll stage), August 21 (boll opening stage) and September 21 (boll opening stage) was measured in each cotton field using a soil moisture recorder (Tengyu® TY-JL/02, Zhengzhou, China).
2.5 Incidence Rate and Disease Index of Verticillium Wilt
A total of 20 continuous cotton samples from each plot were selected to measure the Verticillium wilt incidence rate and disease index. The disease index was divided into five grades based on the extent of Verticillium wilt in cotton plants. Specifically, 0 = no diseased leaves in the cotton plants; 1 = less than 25% diseased leaves; 2 = more than 25% but less than 50% diseased leaves; 3 = more than 50% but less than 75% diseased leaves; 4 = more than 75% diseased leaves [16]. The incidence index was calculated using the following formula:
where i was the disease progression, Xi was the number of cotton plants with a disease progression of i, n was the highest disease progression, and Y was the number of investigated cotton plants.
2.6 Soil DNA Extraction and Amplification
DNA was extracted from 0.5 g aliquots of soil samples using a MN NucleoSpin 96 Soil (Macherey-Nagel Germany) according to the manufacture’s specifications. The V3–V4 hypervariable region of the bacterial 16S rRNA gene was then amplified by PCR using the primers 338F (5′-ACTCCTACGGGAGGCAGCA-3′) and 806R (5′-GGACTACHVGGGTWTCTAAT-3′) [30]. The fungal ITS1 region was amplified using the primers ITS2-F (5′-GCATCGATGAAGAACGCAGC-3′) and ITS2-R (5′-TCCTCCGCTTATTGATATGC-3′) [31]. Following amplification, the samples were sent to BMK (Beijing, China) for sequencing.
2.7 DNA Copy Numbers of V. dahliae in Soil
The copy numbers of V. dahliae DNA were measured by real time fluorescence quantitative PCR (RT-qPCR) using the method described by Zhao et al. [32]. An analysis of each sample was repeated three times.
2.7.1 DNA Extraction, Preparation and Verification of Recombinant Plasmids
The genomic DNA of V. dahliae was extracted via the CTAB method, after which ITS gene sequences were amplified by PCR using the DB19 specific primers (CGGTGACATAATACTGAGAG)/DB20(GACGATGCGGATTGAACGAA). The PCR products were then transformed into the vector pMD19-T (Takara) for 4 h at 16°C, after which they were transformed into Escherichia coli DH5α cells and cultured overnight at 37°C. Next, randomly selected white colonies were transferred to LB liquid medium containing 100 μg·mL−1 ampicillin and cultured overnight at 37°C and 200 rpm. The recombinant plasmid was subsequently extracted from positive clones using a TIAN prep Mini Plasmid Kit (Tiangen Biotech Co., Ltd., Beijing, China). The concentration was then measured on a Nanodrop 2000 spectrophotometer, after which the recombinant plasmid was sent to Sangon Biotech (Shanghai) Co., Ltd. for sequencing. The sequencing results showed that the inserted sequence of the recombinant plasmid was 100% homologous with the ITS gene sequences of V. dahliae.
2.7.2 Establishment of Real-Time Quantitative PCR System and Standard Curve
Plasmid was diluted with a 10-fold gradient and then used as a template for real-time PCR amplification. PCR was performed in the following reaction system: 10 µL of 2 × Master Mix (DBI Bioscience, Germany), 1 µL of primer-F (CCCGCCGGTCCATCAGTCTCTCTG), 1 µL of primer-R (CGGGACTCCGATGCGAGCTGTAAC) [33], 0.4 μL of ROX, 1 μL of template, and ddH2O to 20 µL. The amplification procedure was as follows: denatured at 95°C for 30 s, followed by 40 cycles of 95°C for 5 s and 60°C for 30 s. A standard curve was established with the log value of the plasmid copy number as the horizontal ordinate and the cycle threshold (Ct) as the longitudinal ordinate.
2.7.3 DNA Copy Numbers of V. dahliae
DNA was extracted from different soil samples and used as a template for real-time PCR. The analysis of each sample was repeated three times to obtain the average Ct values of different soil samples. The DNA copy numbers of V. dahliae in the soil were calculated based on the above standard curve.
Raw sequences were processed using FLASH (version 1.2.11) [34], and the quality of the reads was filtered using Trimmomatic (version 0.33) [35]. Chimeric sequences were detected and removed using the USEARCH program (version 8.1) [36], which yielded high-quality tags. The sequences were clustered at the 97% similarity level using USEARCH (version 10.0) [37], with 0.005% of all high-quality tags sequences as the threshold to filter OTUs [38]. The phyla and families of bacteria were identified using the Silva reference database (http://www.arb-silva.de) [39] with the RDP classifier (version 2.2, https://anaconda.org/bioconda/rdp_classifier) [40] and a confidence threshold of 0.8. The phyla and families of fungi were identified using the Unite reference database (http://unite.ut.ee/index.php) [41].
One-way analysis of variance (ANOVA) and the multiple comparisons test were conducted using SPSS 21.0 (IBM Inc., Chicago, IL, USA). The number of OTUs and microbial diversity were further analyzed by post-hoc comparisons using Duncan’s new multiple range test. Different species between CK and RTL were identified by Metastats analysis based on a p < 0.05 [42]. Principal coordinate analysis (PCoA) based on the Bray–Curtis distances and permutation multivariate analysis of variance (PERMANOVA) were used to evaluate differences in the microbial community structure. Redundancy analysis was conducted by Vegan package in R. PICRUSt2 [43] based on KEGG database and Bugbase [44] was used to predict 16S rRNA gene sequences.
3.1 Cotton Yield, Incidence Rate and Disease Index of Verticillium Wilt
Compared to CK, the cotton yield increased 9.19% after RTL treatment (Table 1). In the CK treatment, the incidence rate of Verticillium wilt in cotton fields was as high as 87.50%, while it decreased to 62.50% in the RTL treatment. When compared to CK, the disease index of Verticillium wilt in cotton fields decreased by 42.64% in the RTL treatment (Table 1, Fig. S3). These results showed that RTL can simultaneously decrease the incidence rate and disease index of cotton Verticillium wilt.
3.2 Soil Physical and Chemiacl Properties
Compared to CK, the soil physical properties changed significantly in response to RTL treatment (Table 2). Soil bulk density decreased by 4.12% and 3.55% in the 20–40 cm soil layer in the RTL treatment in June and August, respectively. Total soil porosity increased by 8.78% and 8.71% significantly in the 20–40 cm soil layer in August, while capillary porosity decreased by 7.31% and 4.66% in June and decreased by 6.27% and 6.74% in August, respectively. Non-capillary porosity increased by 30.50% and 101.47% in the 0–20 and 20–40 cm soil layer in June and increased by 21.14% and 104.52% in August, respectively.
Soil moisture content was measured from April to September (Fig. 1). When compared to CK, the moisture content of the 0–20 and 20–40 cm layers increased by 23.40% and 24.00%, 18.10% and 9.30%, and 22.10% and 13.10% in the RTL treatment at the bud stage (6/20), full blooming stage (7/20) and boll opening stage (9/21), respectively. The effects of RTL treatment on moisture content in the topsoil (0–20 cm) were greater than in the subsoil (20–40 cm). Overall, soil moisture content was increased in response to RTL treatment, which may have been related to changes in soil porosity.
Figure 1: Soil moisture content of cotton fields
The average soil temperature at the same soil depth did not differ significantly between CK and RTL. In August, the average soil temperature at 30 cm was lower than that at 10 cm (Table 3). Additionally, the daily temperature range increased at 10 cm in both June and August.
3.3 Taxonomic Characterization of Soil Microbiota
After quality filtering, a total of 1,705,825 bacterial 16S rRNA reads (ranging from 68,746 to 73,951 per sample) (Tables S1, S2) and 1,568,465 fungal ITS reads (ranging from 62,745 to 70,146 per sample) were obtained from the 24 analyzed soil samples (Tables S1, S3). All of the sequences clustered into 2257 bacterial and 1358 fungal OTUs with 97% similarity (Table S4).
Bacterial analysis revealed no significant differences in the number of OTUs between RTL and CK during June, but the number of OTUs increased significantly in the subsoil in August (Fig. S4A). Comparison of the number of OTUs among soil layers revealed that, under CK, the number of OTUs in the subsoil was significantly lower than that in the topsoil in August, but there was no significant difference in the number of OTUs in the two layers of soil under RTL. From June to August, the number of OTUs in topsoil remained stable in CK, but decreased significantly in the subsoil. In RTL, the number of OTUs in topsoil increased significantly, while the number of OTUs in subsoil showed no significant difference.
There was no significant difference in the number of fungal OTUs between CK and RTL in June (Fig. S4B), but the number of OTUs in the 20–40 cm layer was significantly higher than that in the 0–20 cm layer. In August, the number of OTUs in the topsoil of the CK treatment was significantly higher than that in the RTL treatment, whereas the number of OTUs in subsoil did not differ significantly between CK and RTL. We also found that the number of OTUs in topsoil was significantly higher in August than June, while that it tended to be uniform between topsoil and subsoil in August.
3.4 Changes in Microbial Diversity and Composition
We further evaluated the microbial diversity of all soil samples based on the Chao1 index and the Shannon index (Table 4). The Chao1 and Shannon indexes of bacteria in June did not differ significantly between CK and RTL. However, these indexes both increased in subsoil after RTL in August, indicating that RTL t significantly enhanced bacterial richness and evenness in subsoil relative to CK. Moreover, the results suggested that the alpha-diversity of bacteria changed over time.
When compared with the 20–40 cm soil layer in the CK treatment, the Chao1 and Shannon indexes of the bacterial community increased significantly in the 0–20 cm soil layer in the RTL treatment in August; however, there was no significant difference in June. Comparison of samples from different soil depths in the same treatment revealed that the Shannon index of subsoil was higher than that of topsoil after RTL, while there was no significant difference between soil depths in CK in June. The Chao1 and Shannon indexes became more uniform between topsoil and subsoil after RTL in August.
Evaluation of the fungal community revealed no significant change in the Chao1 and Shannon index after RTL, except for samples collected from the 0–20 cm soil layer during June (Table 4). However, the RTL treatment decreased the variation in fungal evenness and richness between the topsoil and subsoil. Comparison of topsoil from CK with subsoil from RTL revealed that the Chao1 index increased significantly after RTL in June, while the Shannon index decreased significantly after RTL in August. Comparison of subsoil from CK with topsoil from RTL revealed that the Chao1 and Shannon indexes did not change significantly in June or August. Taken together, these results indicated that the bacterial and fungal diversity responded differently to RTL. PCoA based on the Bray–Curtis distance was conducted to evaluate the microbial community composition of soil samples subjected to different tillage practices (Fig. 3). For bacteria, PCoA1 and PCoA2 explained 43.03% and 15.12% of the bacterial community composition variance, respectively (Fig. 2A). Moreover, PCoA showed that samples in the CK and RTL treatments fell into different groups, indicating that RTL could change the microbial community composition. Samples from the 20–40 cm layer in the RTL treatment were clustered with samples from the 0–20 cm layer in CK in June and August. Samples collected from the 0–20 cm layer in the RTL treatment and the 20–40 cm layer in CK in August formed two groups. When combined with the alpha diversity data, these findings suggested that the community composition changed significantly after moving soil from 20–40 to 0–20 cm.
Figure 2: Principal co-ordinate analysis (PCoA) of bacterial and fungal communities. PCoA based on Bray–Curtis distance. (A) PCoA of the bacterial community derived from June and August; (B) PCoA of the fungal community derived from June and August
Figure 3: Relative abundance of microbial community. The top 10 and top 15 families are shown in this figure. The remaining families were merged in “Others”, “Unknown” and “Unclassified” are those that were not taxonomically annotated. (A) Top 10 most abundant of bacterial phyla in the 0–20 and 20–40 cm soil layers; (B) top 10 most abundant of fungal phyla in the 0–20 and 20–40 cm soil layers; (C) top 15 most abundant of bacterial families in 0–20 and 20–40 cm soil layers; (D) top 15 most abundant of fungal families in 0–20 and 20–40 cm soil layers
Among fungi, PCoA1 and PCoA2 contributed 34.68% and 19.49% of the total variation, respectively (Fig. 2B). PCoA indicated that the samples were mainly divided into three groups. Samples from the 0–20 cm layer in June in CK and RTL were clustered together, as were samples from the 20–40 cm layers in CK and RTL; however, samples from June and August were divided into two groups. These results suggested that the fungal community composition changed with the original soil layer and was affected by the soil layer depth and cotton growth stage.
The dominant phyla (top 10) identified from the bacterial 16s rRNA genes were the same across both treatments and soil depths (Proteobacteria, Acidobacteria, Actinobacteria, Gemmatimonadetes, Choroflexi, Rokubacteria, Planctomycetes, Bacteroidetes, Nitrospirae and Verrucomicrobia) (Fig. 3A). However, the relative abundance of the dominant phyla varied among treatments. For example, the relative abundance of Proteobacteria in the RTL treatment was higher than that in CK at 0–20 and 20–40 cm. The dominant families (top 15) which can be recognized was 8 in both soil layers, Gemmatimonadaceae, Pyrinomonadaceae, Nitrosomonadaceae, Sphingomonadaceae, Nitrospiraceae, bacteriap25 and Soilbacteraceae_subgrop3 and A4b (Fig. 3C). When compared with CK, the relative abundance of Sphingobacteriaceae and Nitrospiraceae increased significantly, while that of Pyrinomonadaceae decreased significantly in the topsoil after RTL treatment in June. In August, the relative abundance of Nitrosomonadaceae and Pyrinomonadaceae in the topsoil changed significantly after RTL treatment in topsoil, while that of Sphingomonadaceae, Gemmatimonadaceae and Nitrospiraceae in the subsoil changed significantly after RTL. Among these organisms, the abundance of Sphingomonadaceae increased, whereas that of all other species decreased (Table S5).
The dominant phyla (top 10) identified for the fungal ITS2 gene across both treatments and soil depths are shown in Fig. 3B. The relative abundance of Ascomycota, Mortierellomycota and Basidiomycota represented more than half of the total, ranging from 67.6% to 92.7% in the different treatments. The dominant families (top 15) in both soil layers were Mortierellaceae, Aspergillaceae, Nectriaceae, Cladosporiaceae, Chaetomiaceae, Plectosphaerellaceae, Mycosphaerellaceae, Debaryomycetaceae, Stachybotryaceae, Lasiosphaeriaceae, Hypocreales_fam_Incertae_sedis, Bulleribasidiaceae, Pleosporaceae, Clavicipitaceae and Cordycipitaceae (Fig. 3D). In June, the relative abundance of Pleosporaceae, Plectosphaerellaceae, Clavicipitaceae, Aspergillaceae and Mortierellaceae changed significantly after RTL treatment compared with CK. Specifically, the abundance of Pleosporaceae increased in topsoil, whereas that of Plectosphaerellaceae and Clavicipitaceae decreased. Additionally, the abundance of Aspergillaceae increased in subsoil, while that of Mortierellaceae decreased. The abundance of Chaetomiaceae, Lasiosphaeriaceae and Plectosphaerellaceae also changed significantly after RTL treatment in August, with that of Lasiosphaeriaceae decreasing in the topsoil and that of Chaetomiaceae and Plectosphaerellaceae increasing in the subsoil (Table S6).
Metastats analysis showed that, the relative abundance of V. dahliae decreased significantly after RTL in the 0–20 cm soil layer in June, but increased in the 20–40 cm soil layer. In August, the relative abundance of V. dahliae did not differ significantly between CK and RTL (Table 5). RT-PCR showed that the abundance of V. dahliae decreased after RTL in the 0–20 cm soil layer in both June and August, while there was no significant difference between CK and RTL in the 20–40 cm layer (Fig. 4). The change in V. dahliae in topsoil after RTL may partially explain for the decline in the incidence rate and index of cotton Verticillium wilt that was observed in this study.
Figure 4: RT-PCR of genes of Verticillium dahliae. Different letters on different columns indicate a significant difference at p < 0.05
Sequencing of the 16S gene revealed the presence of biocontrol bacteria, such as Bacillus, Pseudomonas and Pseudoxanthomonas (Table 6). The relative abundance of Bacillus and Pseudomonas, which are often reported as efficient biocontrol agents, increased by 61.24% and 887.22% in topsoil, respectively, while it decreased by 63.77% and 87.98% in subsoil after RTL treatment. Pseudoxanthomonas can inhibit root knot nematodes, which can cause plant root wounds and promote the occurrence of soil borne diseases [45,46]. The relative abundance of Pseudoxanthomonas increased more than 6 times and 14 times in June and August after RTL treatment, respectively, in the 0–20 cm soil layer.
To investigate the function differences between CK and RTL treatment, we performed a functional analysis of microbiota using Pircust2 (Tables S7–S10). There had no significant difference between CK and RTL in June, and the top 3 pathway is Metabolic pathway, Biosynthesis of secondary metabolites and Biosynthesis of antibiotics (Tables S7, S8). ABC transporters and Quorum sensing are more active in 0–20 cm soil layer in August after RTL (Fig. 5, Table S9). Bugbase phenotype analysis showed that aerobic, facultatively anaerobic, gram positive, stress tolerant and contains mobile element increased in after RTL in August (Fig. 6).
Figure 5: Predictive analysis of CK and RTL microbial function. The differential analysis of metabolic pathways based on the KEGG database, Light blue represent CK treatment and light purple represent RTL treatment. The right ordinate is the corrected p-value and the left ordinates are different pathway labels
Figure 6: Phenotype prediction of bacterial community in CK and RTL by Bugbase in August. Asterisk indicate a significant difference
Redundancy analysis (RDA) was conducted to investigate the relationship between the relative abundance of V. dahliae, Bacillus, Pseudomonas and Pseudoxanthomonas and soil physical properties in August (Fig. 7). A Monte Carlo test showed that the soil bulk density (SBD), total soil porosity (TSP), non-capillary porosity (NCP) and capillary porosity (CP) were significantly correlated with changes in soil pathogens and biocontrol bacteria (Table S11). Specifically, V. dahliae and Pseudoxanthomonas were correlated with SBD and TSP, while Bacillus and Pseudomonas were correlated with NCP and CP. Additionally, Bacillus were negatively correlated with V. dahliae. The relationships between the relative abundance of V. dahliae, Bacillus, Pseudomonas and Pseudoxanthomonas and soil physical properties of soil samples from June were also analyzed (Fig. S5). Environmental factors were not significantly correlated in June (Table S12).
Figure 7: Redundancy analysis (RDA) of the relationship between microbial communities and soil properties of samples in August. SMC: soil moisture content, SBD: soil bulk density, TSP: total soil porosity, NCP: non-capillary porosity, AT: average temperature
4.1 Effects of Restructuring Tilth Layers on Soil Physical Properties
In this study, RTL was found to increase soil total porosity and non-capillary porosity (Table 2), which was consistent with the results of previous studies [47]. Soil porosity is one of the factors that influences microbial communities [48]. Bugbase phenotype analysis suggesting that aerobic function increased and anaerobic function decreased after RTL, therefore, we speculate that the improvement of the total soil porosity and noncapillary porosity increased the soil permeability and may have enhanced the proportion of aerobic microorganisms, thereby affecting the composition of microbial communities. RDA and a Monte Carlo permutation test showed that soil bulk density, total soil porosity, non-capillary porosity and capillary porosity were significantly correlated with changes in V. dahliae and biocontrol bacteria in August, suggesting that the changes in soil physical properties may affect the relative abundance of V. dahliae, Bacillus, Pseudomonas and Pseudoxanthomonas. Previous research showed that soil bulk density was the dominant environmental factors impacting soil pathogens [49], indicating that the decrease in soil bulk density after RTL treatment may had an inhibitory impact on the relative abundance of V. dahliae. However, it was not clear soil bulk density regulated the relative abundance of V. dahliae directly or indirectly. Further explorations is required to determine whether there is a complex network regulatory relationship between physical properties (e.g., soil bulk density and soil porosity) and V. dahliae and biocontrol bacteria.
4.2 Effects of Restructuring Tilth Layers on Microbial Diversity and Community Composition
The soil microbial community is an important indicator of soil quality and tillage impacts soil microbes [4]. In this study, RTL exchanged the subsoil with the topsoil, after which the microbial groups of the different soil depths changed accordingly. The Chao1 and Shannon indexes indicated that RTL treatment increased the alpha-diversity of the bacterial communities in subsoil in August (Table 4). Meanwhile, functional predication analysis suggesting that the stress tolerant of bacterial community enhanced in August. The diversity of soil microbial communities can be essential to the capacity of soils to suppress soil-borne plant pathogens, with a higher microbial diversity resulting in more competitors and antagonists that soil borne diseases must compete with [50]. Accordingly, increased bacterial diversity may play a positive role in inhibition of Verticillium wilt. In this study, we compared samples from original soil depths with those from exchanged soil depths (CK-topsoil vs. RTL-subsoil, CK-subsoil vs. RTL-topsoil). Although we found no significant changes in the Chao1 and Shannon indexes after replacement of the subsoil with topsoil, they both increased after replacement of the topsoil with subsoil. These results indicated that the soil microbial diversity could be rapidly adjusted after the transfer of subsoil to topsoil, while the soil microbial diversity remained unchanged or changed slowly. Notably, these changes were not observed in samples collected in June, possibly because there was no difference in the alpha-diversity of samples from different soil depths at this time. The Chao1 index of the fungal community increased after RTL in the 0–20 cm soil layer in June relative to CK (Table 5), while fungal variance of diversity between the topsoil and subsoil decreased in August, indicating that the community distribution had become more uniform. However, a difference in bacterial diversity between CK and RTL emerged in August. Taken together. these results suggest that three fungal community responded to treatment faster than the bacterial community.
PCoA showed that samples in CK and RTL could be divided into different groups (Fig. 3A), indicating that the bacterial community composition changed after RTL. Samples from the 0–20 cm layer in CK and the 20–40 cm layer in RTL were clustered, but samples from the 20–40 cm in CK and the 0–20 cm layer in RTL were divided into two groups, demonstrating that the bacterial community composition changed greatly after soil was transferred from 20–40 to 0–20 cm. Analysis of the fungal community revealed that samples from the same soil depth for CK and RTL were clustered together, except for those collected from the 0–20 cm layer in August (Fig. 3B). Specifically, the fungal community composition at 0–20 cm differed between CK and RTL in August, while the others showed no difference. Samples of the original soil layers and the replaced soil layers (0–20 cm soil layer in CK and 20–40 cm soil layer in RTL, 20–40 cm soil layer in CK and 0–20 cm soil layer in RTL) were also not clustered together, indicating that fungal community composition changed after exchange of topsoil and subsoil. Soil microbial diversity and community composition not only play important roles in soil quality, but also in the sustainable development of soil [51]. Reduced biodiversity weakens the functionality and productivity of soil ecosystems, because the number and type of species in the soil ecosystem are responsible for the ecosystem’s overall functional activities [52]. Therefore, soil with higher diversity is more resistant to stresses and protected plants against soil-borne diseases [53], and the change in community composition after RTL treatment may have been associated with the decrease in Verticillium wilt.
RTL did not change the dominant soil bacterial and fungal phyla or families (Fig. 5), which agrees with the results of previous research [54,55]. These findings suggest that these communities are likely dominated by taxa that have adapted to the environmental conditions or that a stable dynamic balance mode was formed after long-term manual management [56]. However, the relative abundance of microbial communities changed significantly after RTL. Gemmatimonadaceae have been found have a biofertilization function [57], while Nitrosomonadaceae and Nitrospiraceae have been reported to be involved in nitrification and denitrification [58], therefor, these organisms may have influences the changes in soil nutrient concentration that occurred after RTL.
4.3 Effects of Restructuring Tilth Layers on Soil-Borne Pathogens and Biocontrol Micro-Bacteria
We found that RTL decreased the incidence rate and incidence index of cotton Verticillium wilt. Previous research showed that deep tillage, which is similar to RTL, reduced the incidence of Verticillium wilt in soil and that the occurrence of wilt was less frequent in deep tillage fields than in conventional cotton fields [59]. Additionally, ITS sequencing and RT-PCR showed that the abundance of V. dahliae decreased in topsoil. Pervious research demonstrated that the final severity of Verticillium wilt was positively correlated with the density of V. dahliae [60]; therefore the reduced incidence of Verticillium wilt may be related with the decreased abundance of V. dahliae that was observed in the present study.
The relative abundance of biocontrol bacteria increased significantly in the 0–20 cm soil layer. Li et al. [61] found that Bacillus exerts excellent biological control of soil-borne diseases, including Verticillium wilt. In the present study, redundancy analysis showed that Bacillus was negatively correlated with V. dahliae, indicating that Bacillus may have inhibited the multiplication of V. dahlia, and therefore be related to the decrease in Verticillum wilt. The relative abundance of V. dahliae and Pseudoxanthomonas was positively correlated (Fig. 6). As mentioned above, root knot nematodes can cause plant root wounds and promote the occurrence of soil-borne diseases [45,46]. Recent research has shown that Pseudoxanthomonas has strong nematostatic activity against root-knot nematodes on tomatoes [62]. The relationship between V. dahliae and Pseudoxanthomonas showed that Pseudoxanthomonas may have repressed the infection of cotton by V. dahliae by controlling root-knot nematodes, thereby reducing the occurrence of Verticillium wilt. Pseudomonas spp. have long been known to confer disease suppression through multiple mechanisms [63]. For example, Pseudomonas inhibits soil-borne pathogens via the production of a wide spectrum of bioactive metabolites [64]. However, there was almost no correlation observed between Pseudomonas and V. dahliae in this study. These findings suggest that the increase of Bacillus and Pseudoxanthomonas were related to the decrease of Verticillium wilt in cotton fields. However, further study is needed to elucidate the mechanism through which Bacillus and Pseudoxanthomonas decrease Verticillium wilt.
The tillage effect on soil bacteria showed a certain temporal dependency and its effects on soil fungi became more consistent over time. We conducted a one-year investigation of RTL in this study. After RTL implementation, the soil structure may gradually return to the previous structure over the next few years or change in other ways. In future studies, we intend to investigate these aspects of the soil environment after RTL implementation.
Acknowledgement: We thank Xihai Liu for the management and assistance in field trial.
Funding Statement: This research was supported by the Basic Research Funds of Hebei Academy of Agriculture and Forestry Sciences (2021070201), Natural Science Foundation of Hebei Province (C2019301097) and China Agriculture Research System-Cotton (CARS-15-18).
Author Contributions: The authors confirm contribution to the paper as follows: data analysis and drafting of the manuscript: Ming Dong; data collection and sample collection: Yan Wang; design and conducting the experiment: Shulin Wang; acquisition of the funding: Guoyi Feng; assistance designing the experiment: Qian Zhang; Yongzeng Lin; assistance conductiong the experiment: Qinglong Liang; revision of the manuscript: Yongqiang Wang; acquisition of funds and manuscript revision: Hong Qi. All authors reviewed the results and approved the final version of the manuscript.
Availability of Data and Materials: The raw data of bacterial 16S rRNA and fungal ITS sequence data were deposited in the NCBI Sequence Read Archive (SRA) database under accession number PRJNA924506 (http://www.ncbi.nlm.nih.gov/bioproject/924506) and PRJNA924532 (http://www.ncbi.nlm.nih.gov/bioproject/924532), respectively.
Ethics Approval: Not applicable.
Conflicts of Interest: The authors declare that they have no conflicts of interest to report regarding the present study.
Supplementary Materials: The supplementary material is available online at https://doi.org/10.32604/phyton.2023.030465.
References
1. Hobbs, P. R., Sayre, K., Gupta, R. (2008). The role of conservation agriculture in sustainable agriculture. Philosophical Transactions of the Royal Society B: Biological Sciences, 363(1491), 543–555. [Google Scholar]
2. Zhang, Z., Peng, X. (2021). Bio-tillage: A new perspective for sustainable agriculture. Soil and Tillage Research, 206, 104844. [Google Scholar]
3. Acharya, C. L., Sharma, P. D. (1994). Tillage and mulch effects on soil physical environment, root growth, nutrient uptake and yield of maize and wheat on an Alfisol in North-West India. Soil and Tillage Research, 32(4), 291–302. [Google Scholar]
4. Dai, H., Zhang, H., Li, Z., Liu, K., Zamanian, K. (2021). Tillage practice impacts on the carbon sequestration potential of topsoil microbial communities in an agricultural field. Agronomy, 11(1), 60. [Google Scholar]
5. Wang, S. L., Wang, H., Li, J., Lyu, W., Chen, N. N. et al. (2016). Effects of long-term straw mulching on soil organic carbon, nitrogen and moisture and spring maize yield on rain-fed croplands under different patterns of soil tillage practice. The Journal of Applied Ecology, 27(5), 1530–1540. [Google Scholar]
6. Degrune, F., Theodorakopoulos, N., Colinet, G., Hiel, M. P., Bodson, B. et al. (2017). Temporal dynamics of soil microbial communities below the seedbed under two contrasting tillage regimes. Frontiers in Microbiology, 8, 1127. [Google Scholar] [PubMed]
7. Sun, R., Li, W., Dong, W., Tian, Y., Hu, C. et al. (2018). Tillage changes vertical distribution of soil bacterial and fungal communities. Frontiers in Microbiology, 9, 699. [Google Scholar] [PubMed]
8. Riley, H., Pommeresche, R., Eltun, R., Hansen, S., Korsaeth, A. (2008). Soil structure, organic matter and earthworm activity in a comparison of cropping systems with contrasting tillage, rotations, fertilizer levels and manure use. Agriculture, Ecosystems & Environment, 124(3), 275–284. [Google Scholar]
9. Zhai, L., Xu, P., Zhang, Z., Li, S., Xie, R. et al. (2017). Effects of deep vertical rotary tillage on dry matter accumulation and grain yield of summer maize in the Huang-Huai-Hai Plain of China. Soil and Tillage Research, 170, 167–174. [Google Scholar]
10. Ahl, C., Joergensen, R. G., Kandeler, E., Meyer, B., Woehler, V. (1998). Microbial biomass and activity in silt and sand loams after long-term shallow tillage in central Germany. Soil and Tillage Research, 49(1), 93–104. [Google Scholar]
11. Mu, X., Zhao, Y., Liu, K., Ji, B., Guo, H. et al. (2016). Responses of soil properties, root growth and crop yield to tillage and crop residue management in a wheat-maize cropping system on the North China Plain. European Journal of Agronomy, 78, 32–43. [Google Scholar]
12. Zhang, L., Wang, J., Fu, G., Zhao, Y. (2018). Rotary tillage in rotation with plowing tillage improves soil properties and crop yield in a wheat-maize cropping system. PLoS One, 13(6), e0198193. [Google Scholar] [PubMed]
13. Wang, S., Qi, H., Wang, Y., Zhang, Q., Feng, G. et al. (2017). Effects of restructuring tilth layer in soil physical and chemical properties and cotton development in continuous cropping cotton fields. Acta Agronomica Sinica, 43(5), 741–753. [Google Scholar]
14. Wang, S., Qi, H., Wang, Y., Zhang, Q., Feng, G. et al. (2018). Effects of restructuring tilth layer and irrigation on soil water content and cotton yield traits. Journal of China Agricultural University, 23(1), 27–36. [Google Scholar]
15. Wang, S., Wang, Y., Qi, H., Zhang, Q., Feng, G. et al. (2017). Study of restructuring soil layers on weeds control in cotton fields. Journal of Shanxi Agricutural University, 37(4), 235. [Google Scholar]
16. Li, P., Wang, S., Qi, H., Wang, Y., Zhang, Q. et al. (2019). Soil replacement combined with subsoiling improves cotton yields. Journal of Cotton Research, 2(1), 25–40. [Google Scholar]
17. Yao, Y. W., Fu, C. Z., Wang, W. L., Li, Q., Zhang, Y. N. et al. (1982). Preliminary studies on physiological forms of cotton Verticillium wilt fungus. Acta Phytophylacica Sin, 9, 145–148. [Google Scholar]
18. Fradin, E. F., Thomma, B. P. H. J. (2006). Physiology and molecular aspects of Verticillium wilt diseases caused by V. dahliae and V. albo-atrum. Molecular Plant Pathology, 7(2), 71–86. [Google Scholar] [PubMed]
19. Inderbitzin, P., Subbarao, K. V. (2014). Verticillium systematics and evolution: How confusion impedes verticillium wilt management and how to resolve it. Phytopathology, 104(6), 6564–6574. [Google Scholar]
20. Harris, D. C., Yanf, J. R. (2010). The relationship between the amount of Verticillium dahliae in soil and the incidence of strawberry wilt as a basis for disease risk prediction. Plant Pathology, 45(1), 106–114. [Google Scholar]
21. Wei, F., Fan, R., Dong, H., Shang, W., Xu, X. et al. (2014). Threshold microsclerotial inoculum for cotton verticillium wilt determined through wet-sieving and real-time quantitative PCR. Phytopathology®, 105(2), 220–229. [Google Scholar]
22. Karademir, E., Karademir, C., Ekinci, R., Baran, B., Sagir, A. (2012). Effect of Verticillium dahliae Kleb. on cotton yield and fiber technological properties. International Journal of Plant Production, 6(4), 387–407. [Google Scholar]
23. Robb, J. (2007). Verticillium tolerance: Resistance, susceptibility, or mutualism? Canadian Journal of Botany, 85(10), 903–910. [Google Scholar]
24. Wei, F., Feng, H., Zhang, D., Feng, Z., Zhao, L. et al. (2021). Composition of rhizosphere microbial communities associated with healthy and verticillium wilt diseased cotton plants. Frontiers in Microbiology, 12, 618169. [Google Scholar] [PubMed]
25. Compant, S., Duffy, B., Nowak, J., Clément, C., Barka, E. A. (2005). Use of plant growth-promoting bacteria for biocontrol of plant diseases: Principles, mechanisms of action, and future prospects. Applied and Environmental Microbiology, 71(9), 4951–4959. [Google Scholar] [PubMed]
26. Yang, Y., Fang, J., Ma, W., Guo, D., Mohammat, A. (2010). Large-scale pattern of biomass partitioning across China’s grasslands. Global Ecology and Biogeography, 19(2), 268–277. [Google Scholar]
27. Shidan, B. (2011). Soil and agricultural chemistry analysis. Beijing, China: China Agricultural Press. [Google Scholar]
28. Heiskanen, J. (1992). Comparison of three methods for determining the particle density of soil with liquid pycnometers. Communications in Soil Science and Plant Analysis, 23(7–8), 841–846. [Google Scholar]
29. Sheng, M., Xiong, K., Wang, L., Li, X., Li, R. et al. (2018). Response of soil physical and chemical properties to Rocky desertification succession in South China Karst. Carbonates and Evaporites, 33(1), 15–28. [Google Scholar]
30. Xu, H., Chen, Z., Wu, X., Zhao, L., Wang, N. et al. (2021). Antibiotic contamination amplifies the impact of foreign antibiotic-resistant bacteria on soil bacterial community. Science of the Total Environment, 758(Suppl. 1), 143693. [Google Scholar] [PubMed]
31. Liu, Y., Zhang, Y., Shi, Y., Shen, F., Yang, Y. et al. (2021). Characterization of fungal aerosol in a landfill and an incineration plants in Guangzhou, Southern China: The link to potential impacts. Science of the Total Environment, 764(3), 142908. [Google Scholar] [PubMed]
32. Zhao, W., Guo, Q., Li, S., Wang, P., Dong, L. et al. (2021). Effects of Bacillus subtilis NCD-2 and broccoli residues return on potato Verticillium wilt and soil fungal community structure. Biological Control, 159, 104628–104637. [Google Scholar]
33. Xiao, R., Yu, Z., Elsharawy, A., Wei, F., Yang, J. (2011). SYBR Green I real time RT-PCR assay for quantitatively detecting the occurrence of Verticillium dahliae of cotton in naturally infested soil. Mycosystema, 30(4), 598–603. [Google Scholar]
34. Magoč, T., Salzberg, S. L. (2011). FLASH: Fast length adjustment of short reads to improve genome assemblies. Bioinformatics, 27(21), 2957–2963. [Google Scholar] [PubMed]
35. Bolger, A. M., Lohse, M., Usadel, B. (2014). Trimmomatic: A flexible trimmer for Illumina sequence data. Bioinformatics, 30(15), 2114–2120. [Google Scholar] [PubMed]
36. Edgar, R. C. (2013). UPARSE: Highly accurate OTU sequences from microbial amplicon reads. Nature Methods, 10(10), 996–998. [Google Scholar] [PubMed]
37. Edgar, R. C., Haas, B. J., Clemente, J. C., Quince, C., Knight, R. (2011). UCHIME improves sensitivity and speed of chimera detection. Bioinformatics, 27(16), 2194–2200. [Google Scholar] [PubMed]
38. Bokulich, N. A., Subramanian, S., Faith, J. J., Gevers, D., Gordon, J. I. et al. (2013). Quality-filtering vastly improves diversity estimates from Illumina amplicon sequencing. Nature Methods, 10(1), 57–59. [Google Scholar] [PubMed]
39. Quast, C., Pruesse, E., Yilmaz, P., Gerken, J., Schweer, T. et al. (2012). The SILVA ribosomal RNA gene database project: Improved data processing and web-based tools. Nucleic Acids Research, 41(D1), D590–D596. [Google Scholar] [PubMed]
40. Wang, Q., Garrity, G. M., Tiedje, J. M., Cole, J. R. (2007). Naive Bayesian classifier for rapid assignment of rRNA sequences into the new bacterial taxonomy. Applied and Environmental Microbiology, 73(16), 5261–5267. [Google Scholar] [PubMed]
41. Kõljalg, U., Nilsson, R. H., Abarenkov, K., Tedersoo, L., Taylor, A. F. S. et al. (2013). Towards a unified paradigm for sequence-based identification of fungi. Molecular Ecology, 22(21), 5271–5277. [Google Scholar]
42. Leamer, E. E. (1978). Specification searches: Ad hoc inference with nonexperimental data. New York: Wiley. [Google Scholar]
43. Bai, S., Hou, G. (2020). Microbial communities on fish eggs from Acanthopagrus schlegelii and Halichoeres nigrescens at the XuWen coral reef in the Gulf of Tonkin. PeerJ, 7(8), e8517. [Google Scholar]
44. Liang, Z., Dong, C. B., Liang, H., Zhen, Y. X., Zhou, R. L. et al. (2022). A microbiome study reveals the potential relationship between the bacterial diversity of a gymnastics hall and human health. Scientific Reports, 12(1), 5663. [Google Scholar] [PubMed]
45. Caillaud, M. C., Dubreuil, G., Quentin, M., Perfus-Barbeoch, L., Lecomte, P. et al. (2008). Root-knot nematodes manipulate plant cell functions during a compatible interaction. Journal of Plant Physiology, 165(1), 104–113. [Google Scholar] [PubMed]
46. Palomares-Rius, J. E., Castillo, P., Navas-Cortés, J. A., Jiménez-Díaz, R. M., Tena, M. (2011). A proteomic study of in-root interactions between chickpea pathogens: The root-knot nematode Meloidogyne artiellia and the soil-borne fungus Fusarium oxysporum f. sp. ciceris race 5. Journal of Proteomics, 74(10), 2034–2051. [Google Scholar] [PubMed]
47. Khan, A. R. (1996). Influence of tillage on soil aeration. Journal of Agronomy and Crop Science, 177(4), 253–259. [Google Scholar]
48. Pandey, S. (2015). Variation of soil microbial population in different soil horizons. Journal of Microbiology and Experimentation, 2(2), 00044. [Google Scholar]
49. Li, R., Tao, R., Ling, N., Chu, G. (2017). Chemical, organic and bio-fertilizer management practices effect on soil physicochemical property and antagonistic bacteria abundance of a cotton field: Implications for soil biological quality. Soil and Tillage Research, 167(1–3), 30–38. [Google Scholar]
50. van Bruggen, A. H. C., Semenov, A. M., van Diepeningen, A. D., de Vos, O. J., Blok, W. J. (2006). Relation between soil health, wave-like fluctuations in microbial populations, and soil-borne plant disease management. European Journal of Plant Pathology, 115(1), 105–122. [Google Scholar]
51. Kennedy, A. C., Smith, K. L. (1995). Soil microbial diversity and the sustainability of agricultural soils. Plant and Soil, 170(1), 75–86. [Google Scholar]
52. Loreau, M., Naeem, S., Inchausti, P., Bengtsson, J., Grime, J. P. et al. (2001). Biodiversity and ecosystem functioning: Current knowledge and future challenges. Science, 294(5543), 804–808. [Google Scholar] [PubMed]
53. She, S., Niu, J., Zhang, C., Xiao, Y., Chen, W. et al. (2017). Significant relationship between soil bacterial community structure and incidence of bacterial wilt disease under continuous cropping system. Archives of Microbiology, 199(2), 267–275. [Google Scholar] [PubMed]
54. Chen, X., Hou, F., Wu, Y., Cheng, Y. (2017). Bacterial and fungal community structures in Loess Plateau grasslands with different grazing intensities. Frontiers in Microbiology, 8, 606. [Google Scholar] [PubMed]
55. Nelson, M. B., Berlemont, R., Martiny, A. C., Martiny, J. B. H. (2015). Nitrogen cycling potential of a grassland litter microbial community. Applied and Environmental Microbiology, 81(20), 7012–7022. [Google Scholar] [PubMed]
56. Duan, N., Li, L., Liang, X., Fine, A., Zhuang, J. et al. (2022). Variation in bacterial community structure under long-term fertilization, tillage, and cover cropping in continuous cotton production. Frontiers in Microbiology, 13, 847005. [Google Scholar]
57. Wang, Z., Huang, F., Mei, X., Wang, Q., Song, H. et al. (2014). Long-term operation of an MBR in the presence of zinc oxide nanoparticles reveals no significant adverse effects on its performance. Journal of Membrane Science, 471, 258–264. [Google Scholar]
58. Jiang, Y., Yin, G., Li, Y., Hou, L., Liu, M. et al. (2022). Saltwater incursion regulates N2O emission pathways and potential nitrification and denitrification in intertidal wetland. Biology and Fertility of Soils, 59, 541–553. [Google Scholar]
59. Chen, H., Zhao, S., Zhao, J., Zhang, K., Jiang, J. et al. (2020). Deep tillage combined with biofertilizer following soil fumigation improved chrysanthemum growth by regulating the soil microbiome. MicrobiologyOpen, 9(7), e1045. [Google Scholar] [PubMed]
60. López-Escudero, F. J., Blanco-López, M. A. (2007). Relationship between the inoculum density of Verticillium dahliae and the progress of verticillium wilt of olive. Plant Disease, 91(11), 1372–1378. [Google Scholar]
61. Li, S. Z., Lu, X. U., Ma, P., Gao, S., Liu, X. Z. et al. (2005). Evaluation of biocontrol potential of a bacterial strain NCD-2 against cotton verticillium wilt in field trials. Acta Phytopathol Sinica, 35(5), 451–455. [Google Scholar]
62. Hu, Y., Li, J., Li, J., Zhang, F., Wang, J. et al. (2019). Biocontrol efficacy of Pseudoxanthomonas japonensis against Meloidogyne incognita and its nematostatic metabolites. FEMS Microbiology Letters, 366(2), 1–7. [Google Scholar]
63. Wang Z., Song Y. (2022). Toward understanding the genetic bases underlying plant-mediated “cry for help” to the microbiota. iMeta, 1(1), e8. [Google Scholar]
64. Weller, D. M. (2007). Pseudomonas biocontrol agents of soilborne pathogens: Looking back over 30 years. Phytopathology, 97(2), 250–256. [Google Scholar] [PubMed]
Cite This Article
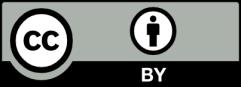