Open Access
ARTICLE
Identification of an immune classifier for predicting the prognosis and therapeutic response in triple-negative breast cancer
1 Department of Breast Surgery, The First Affiliated Hospital of Wenzhou Medical University, Wenzhou, China
2 Department of Visceral, Thoracic and Vascular Surgery, Carl Gustav Carus University Hospital Dresden, Technische Universität Dresden, Dresden, Germany
3 Department of Respiratory and Critical Medicine, The First Affiliated Hospital of Wenzhou Medical University, Wenzhou, China
* Corresponding Author: XIXI LAI. Email:
(This article belongs to the Special Issue: Frontiers in cancer: tumor microenvironment)
BIOCELL 2023, 47(12), 2681-2696. https://doi.org/10.32604/biocell.2023.043298
Received 06 June 2023; Accepted 04 September 2023; Issue published 27 December 2023
Abstract
Objectives: Triple-negative breast cancer (TNBC) poses a significant challenge due to the lack of reliable prognostic gene signatures and an understanding of its immune behavior. Methods: We analyzed clinical information and mRNA expression data from 162 TNBC patients in TCGA-BRCA and 320 patients in METABRIC-BRCA. Utilizing weighted gene coexpression network analysis, we pinpointed 34 TNBC immune genes linked to survival. The least absolute shrinkage and selection operator Cox regression method identified key TNBC immune candidates for prognosis prediction. We calculated chemotherapy sensitivity scores using the “pRRophetic” package in R software and assessed immunotherapy response using the Tumor Immune Dysfunction and Exclusion algorithm. Results: In this study, 34 survival-related TNBC immune gene expression profiles were identified. A least absolute shrinkage and selection operator-Cox regression model was used and 15 candidates were prioritized, with a concomitant establishment of a robust risk immune classifier. The high-risk TNBC immune groups showed increased sensitivity to therapeutic agents like RO-3306, Tamoxifen, Sunitinib, JNK Inhibitor VIII, XMD11-85h, BX-912, and Tivozanib. An analysis of the Search Tool for Interaction of Chemicals database revealed the associations between the high-risk group and signaling pathways, such as those involving Rap1, Ras, and PI3K-Akt. The low-risk group showed a higher immunotherapy response rate, as observed through the tumor immune dysfunction and exclusion analysis in the TCGA-TNBC and METABRIC-TNBC cohorts. Conclusion: This study provides insights into the immune complexities of TNBC, paving the way for novel diagnostic approaches and precision treatment methods that exploit its immunological intricacies, thus offering hope for improved management and outcomes of this challenging disease.Keywords
Supplementary Material
Supplementary Material FileBreast cancer is a multifaceted disease that affects millions of women globally (Siegel et al., 2018) and manifests into diverse subtypes, each characterized by distinct molecular characteristics (Nolan et al., 2023; Testa et al., 2020). Among these subtypes, triple-negative breast cancer (TNBC) has emerged as a perplexing and formidable adversary (Tsang and Tse, 2020; van den Ende et al., 2023), which accounts for approximately 15%–25% of all breast cancer cases and is known for its aggressive behavior and limited treatment options (Almansour, 2022). The proportion of TNBC remains stable across all age groups. However, there are notable variations in its subtypes between younger and older women. Specifically, there is an elevated prevalence of basal-like, apocrine, and neuroendocrine TNBC in younger compared to older women (Hudis and Gianni, 2011). Additionally, African American and Hispanic women are at a higher risk of being diagnosed with TNBC, with a poorer prognosis shown by African American women compared to other ethnic groups (Dietze et al., 2015). Defined by the absence of estrogen receptor (ER), progesterone receptor (PR), and human epidermal growth factor receptor 2 (HER2), TNBC poses significant challenges for researchers and clinicians due to its resistance to conventional therapeutic approaches (Ensenyat-Mendez et al., 2021). Although substantial progress has been made in understanding the genetic alterations and the signaling pathways underlying TNBC, the enigma of its clinical heterogeneity and aggressive nature persists (Derakhshan and Reis-Filho, 2022). Therefore, unraveling the intricate interplay between the tumor and its microenvironment holds immense promise for comprehending the fundamental mechanisms driving TNBC progression and exploring novel therapeutic strategies (Solinas et al., 2017).
The emerging field of the immune microenvironment, a complex milieu comprising immune cells, stromal components, and soluble factors, has garnered particular interest in recent years (Grivennikov et al., 2010). Extensive research has elucidated the pivotal role of the immune system in cancer surveillance and control, including within the context of TNBC (Fan and He, 2022; Park et al., 2018). TNBC and non-TNBC subtypes exhibit distinctive characteristics within the IME, influencing their respective clinical projections (Ding et al., 2023). The unique immunological attributes exhibited by TNBC have sparked intense scrutiny of the interplay between tumor cells and immune cells to decipher the intricate web of interactions that dictate disease outcomes (Romero-Cordoba et al., 2019). TNBC often manifests an IME characterized by the heightened infiltration of immune cell types, such as tumor-infiltrating lymphocytes (TILs) and tumor-associated macrophages (Fan and He, 2022). These infiltrating immune cells mirror the immune response of the host against the tumor and contribute to shaping the immunogenicity and aggressiveness of a tumor (Liu et al., 2011; Miyashita et al., 2015). Furthermore, unraveling the complex molecular crosstalk between tumor cells and immune cells, mediated by cytokines, chemokines, and immune checkpoints, provides a captivating avenue of exploration (Dore-Savard et al., 2016; Lee et al., 2014; Pan et al., 2014; Santoni et al., 2018). The delicate balance between immune activation and evasion within the tumor milieu holds profound implications for devising personalized immunotherapeutic interventions that could revolutionize the TNBC treatment paradigms (Li et al., 2018; Vikas et al., 2018). Previous TNBC classification studies have been conducted to understand the genetic basis and molecular characteristics of TNBC and identify potential prognostic gene signatures (Burstein et al., 2015; Hu et al., 2021b). However, reliable prognostic gene signatures for TNBC immune profiles are currently lacking, and the underlying genetic principles that govern their behavior in this context remain unclear.
The importance of this issue lies in the urgent need for more effective and tailored treatments for TNBC patients. Despite recent advancements in cancer therapeutics, TNBC remains challenging to manage due to its aggressive nature and limited targeted therapy options. Our study’s focus on immune gene expression profiles sheds light on potential prognostic indicators for TNBC and unveils new therapeutic vulnerabilities that can be exploited for personalized treatment strategies. This retrospective study offers valuable insights into the complex immune microenvironment of TNBC, paving the way for innovative approaches to improve patient outcomes and revolutionize TNBC management. Therefore, the two main objectives of this study were to establish a reliable TNBC immune prognostic gene signature for the stratification of patients and to identify potential targets for more effective and individualized therapies. A comprehensive analysis identified 34 survival-related TNBC immune gene expression profiles. A least absolute shrinkage and selection operator (LASSO) Cox risk regression model was employed to prioritize 15 candidate genes, ultimately establishing a robust risk model for TNBC. Additionally, potential chemotherapeutic and immunotherapeutic agents that exhibit sensitivity within specific TNBC immune risk clusters were proposed. By shedding light on the enigma surrounding TNBC, this study aims to pave the way for novel diagnostic approaches and precision treatment methods that exploit the immunological intricacies of this challenging disease.
Acquisition and preprocessing of clinical and gene-expression datasets
The clinical information and mRNA expression data were obtained from the GDC (Genomic Data Commons) portal (https://portal.gdc.cancer.gov/) for The Cancer Genome Atlas—Breast Invasive Carcinoma (TCGA-BRCA) cohort (n = 1,102) in July 2022. The normalized transcriptomic and clinical data of 1980 patients with BRCA in the Molecular Taxonomy of Breast Cancer International Consortium—Breast Cancer (METABRIC-BRCA) cohort were obtained from the cBioPortal (www.cbioportal.org/) for cancer genomics in July 2022. According to the immunohistochemical staining in clinical data, 162 TNBC patients were identified in TCGA-BRCA and 320 in METABRIC-BRCA, defined as TCGA-TNBC and METABRIC-TNBC cohorts.
Using the “xCells” package of the R software, 64 xCells molecular abundance scores for the TCGA-BRCA and METABRIC-BRCA cohorts were obtained (Aran et al., 2017).
ESTIMATE (estimation of stromal and immune cells in malignant tumor tissues using expression data)
The immune, stromal, and environmental scores of the TCGA-BRCA and METABRIC-BRCA cohorts were computed by the “estimate” package of the R software (Yoshihara et al., 2013).
Weighted gene co-expression network analysis (WGCNA) networks
The co-expression networks targeting the multi-xCells abundance scores were identified and constructed by the “WGCNA” package of the R software (Langfelder and Horvath, 2008). Initially, 10000 genes with the highest median absolute deviations were selected as input genes for the TCGA-TNBC and METABRIC-TNBC cohorts individually, and the “mergeCutHeight” parameter was set at 0.5.
The least absolute shrinkage and selection operator Cox regression risk model
The LASSO Cox regression method was employed to prioritize the immune candidates for predicting prognosis using the “glmnet” package of the R software (Tibshirani, 1997; Wilkerson and Hayes, 2010). The formula for the risk score was based on the 15 prioritized candidate genes. The coefficients of the candidates were: APOBEC3C = −0.140184080628171, BTN3A2 = −0.0800840435867073, MS4A7 = 0.692949642926572, SERPING1 = −0.259968579105627, EHD1 = 1.13142714510949, APOBEC3F = −0.117312349404222, IDO1 = −0.0578277242451044, HSD11B1 = −0.2949266494099, MGAT1 = 0.129685497235209, GCA = −0.0401015501719972, CD38 = −0.302456183226881, LAMP3 = −0.0708087440274117, HLA.DOB = −0.0263059953549838, DRAM1 = −0.172912222943017, and TREM2 = 0.0718022672812051.
Differential expression analysis
Differentially expressed mRNAs were calculated by the “edgeR” package of the R software (McCarthy et al., 2012).
Using the “maxstat” method with abseps = 0.01, minprop = 0.3, and maxprop = 0.7, the optimal cutoffs for the LASSO risk scores were determined for the overall survival (OS) in the TCGA-TNBC and METABRIC-TNBC cohorts.
Kaplan-Meier and Univariate Cox regression analysis were performed using the “survival”, “survminer”, and “ggplot2” packages of the R software.
UCSC_TFBS (transcription factor binding sites, university of california, santa cruz) determination
UCSC_TFBS accessions of the differentially expressed mRNAs were determined by the Database for Annotation, Visualization and Integrated Discovery (DAVID) tool (https://david.ncifcrf.gov/tools.jsp) (Huang da et al., 2009; Sherman et al., 2022). The network visualization was constructed by the Cytoscape software (https://cytoscape.org/) (Shannon et al., 2003).
Somatic mutation and copy number variation (CNV) analyses
The top 300 high-frequency somatic mutations in the TCGA-TNBC were accessed from the cBioPortal in July 2022. The chi-square test analyzed the significant enrichment among the distinct prognostic groups.
The global CNV data of the TCGA-BRCA cohort were accessed from the Firebrowse database (http://firebrowse.org/) in January 2022. A segment mean of >0.2 was defined as an amplification, and a segment mean of <−0.2 was defined as a deletion.
The distinct prognostic clusters were identified using Fisher’s exact test and analyzed using the CoNVaQ web tool (https://exbio.wzw.tum.de/convaq/) (Larsen et al., 2018).
The CNV plots were visualized using Integrative Genomics Viewer 2.16.0 (https://software.broadinstitute.org/software/igv/2.16.x) (Robinson et al., 2017).
Gene set enrichment analysis algorithm (GSEA)
A GSEA algorithm was employed in the C5 collection (ontology gene set) of the Molecular Signatures Database (MSigDB), the Hallmark gene set, and the Kyoto Encyclopedia of Genes and Genomes (KEGG) collection terms through the “clusterProfiler” package of the R software (Wu et al., 2021b).
Prediction of the sensitivities of chemotherapy and immunotherapy
The predictions of the chemotherapy sensitivity scores were computed by the “pRRophetic” package of the R software (Geeleher et al., 2014). The predictions of the immunotherapy sensitivity scores in the TCGA-TNBC and METABRIC-TNBC cohorts were performed by using the Tumor Immune Dysfunction and Exclusion (TIDE) algorithm (http://tide.dfci.harvard.edu/).
The search tool for interaction of chemicals (STITCH) analysis
The analyses of the interaction networks between the chemical compounds and the proteins (Szklarczyk et al., 2019) were performed by using the STITCH software (http://stitch.embl.de/).
Single-cell sequencing analysis
The Tumor Immune Single-cell Hub 2 (TISCH2) online database (http://tisch.comp-genomics.org/) (Han et al., 2023) was utilized to analyze and visualize the single-cell sequencing database of 26 cases with BRCA (GSE176078) (Wu et al., 2021a).
We performed all statistical analyses using R version 4.2.3. All statistical tests were two-tailed, and p-values less than 0.05 were considered statistically significant in our study.
Dominant molecular immune subtypes in triple-negative breast cancer
Two independent datasets with 3082 samples, including TCGA-BRCA (n = 1102) and METABRIC-BRCA (n = 1980), were analyzed by ESTIMATE and xCells to assess the abundance of the distinct molecular immune subtypes (Suppl. Fig. S1). Using immunohistochemical staining, 162 TNBC patients were identified in TCGA-BRCA and 320 in METABRIC-BRCA. Compared to the non-TNBC, significantly higher immune and environmental scores and lower stromal scores of TNBC in TCGA-BRCA and METABRIC-BRCA cohorts are indicated (Figs. 1A and 1B, all p-value < 0.001). Of the 64 xCells molecular immune subtypes, 26 were significantly higher (Log2 fold change ≥ 0.6; Wilcoxon p ≤ 0.5) in the TNBC than the non-TNBC cases of the TCGA-BRCA cohort (Suppl. Table S1), and 22 were significantly higher in the TNBC cases of the METABRIC-BRCA cohort (Suppl. Table S2). The 19 alphabetic × Cells molecular immune subtypes were observed to overlap in both TCGA-BRCA and METABRIC-BRCA cohorts (Suppl. Fig. S1).
Figure 1: Estimation of STromal and Immune cells in MAlignant Tumor tissues using Expression data (ESTIMATE) analysis in The Cancer Genome Atlas-Breast Invasive Carcinoma (TCGA-BRCA) as well as Molecular Taxonomy of Breast Cancer International Consortium-Breast Invasive Carcinoma (METABRIC-BRCA) cohorts. Box plots revealing the difference in immune and stroma as well as environment scores between TNBC and non-TNBC in the TCGA-BRCA (A) as well as METABRIC-BRCA cohorts (B).
Establishment of the triple-negative breast cancer immune-related signature
To establish the TNBC immune-related signature, WGCNA was performed in the TCGA-TNBC as well as METABRIC-TNBC cohorts. Ten thousand genes with the highest median absolute deviations were selected as candidates for constructing the WGCNA networks in both cohorts. A scale-free topology network was constructed using the soft thresholding power (β) of eight genes in the TCGA-TNBC cohort (scale-free R2 = 0.95) (Suppl. Fig. S2A) and seven genes in the METABRIC-TNBC cohort (scale-free R2 = 0.97) (Suppl. Fig. S2B) was estimated. Using a dynamic hybrid cutting approach, ten coexpression models were clustered into the selected TCGA-TNBC cohort (Fig. 2A, Suppl. Fig. S3A, and Suppl. Table S3). Notably, the brown module exhibited the strongest positive correlation with almost the dominant molecular immune subtypes of TNBC (Fig. 2C). By again using a dynamic hybrid cutting approach, eight co-expression models were clustered in the METABRIC-TNBC cohort (Fig. 2B, Suppl. Fig. S3B, and Suppl. Table S4). The turquoise module exhibited the strongest positive correlation with almost the dominant molecular immune subtypes (Fig. 2D). In total, 624 and 1367 candidates were clustered in the brown module of the TCGA-TNBC and turquoise module of the METABRIC-TNBC cohorts, respectively, 410 of which overlapped in both modules (Suppl. Fig. S4). Gene Ontology enrichment analysis of the domains of biological processes, cellular components, and molecular functions revealed that T-cell activation, the external side of the plasma membrane, and immune receptor activity were the most crucial individual factors (Suppl. Fig. S5, Suppl. Table S5).
Figure 2: Establishment of triple-negative breast cancer (TNBC) immune related candidate genes based on Weighted Gene Co-Expression Network Analysis (WGCNA). Clustering dendrograms presenting candidates with close expression patterns were clustered into WGCNA modules in The Cancer Genome Atlas- Triple-Negative Breast Cancer (TCGA-TNBC) (A) as well as Molecular Taxonomy of Breast Cancer International Consortium (METABRIC)-TNBC (B) in which gray module genes indicate not assigned in other modules. The correlations between each module eigengenes and phenotypes were presented by heatmaps of module-trait relationships for TCGA-TNBC (C) and METABRIC-TNBC (D).
Construction of a LASSO Cox survival-related TNBC immune prognostic risk model
Of the 410 overlapping candidate genes mentioned above, 34 were significant concerning OS in the TCGA-TNBC cohort based on Univariate Cox analysis (Fig. 3A; p-value < 0.05).
Figure 3: Survival-related triple-negative breast cancer (TNBC) immune signature established by the least absolute shrinkage and selection operator (LASSO) Cox model. Forest plot summarizes 34 overall survival (OS) associated TNBC immune candidates selected by Univariate Cox analysis in TCGA-TNBC (A). The LASSO Cox model revealed the 16 most relevant survival-associated TNBC immune candidates for OS of the TCGA-TNBC cohort (B and C). Kaplan-Meier plots for ten-years OS of the low- and high-risk groups for TCGA-TNBC (D) and METABRIC-TNBC (E), whose strata by the best cutoff algorithm. Log-rank p-values were computed for those two TNBC cohorts. The line graph presents the receiver operating characteristic analysis in TCGA-TNBC (F).
In the TCGA-TNBC cohort, the 15 most relevant survival-associated TNBC immune genes were prioritized concerning OS by a LASSO Cox regression risk model (Figs. 3B and 3C). Among the analyzed genes, four showed associations with unfavorable OS, while 11 exhibited associations with favorable OS.
The high- and low-risk groups were stratified according to the best cutoff of the LASSO Cox risk score by using log-rank analysis in the TCGA-TNC (Fig. 3D; p-value < 0.0001) and METABRIC-TNBC cohorts (Fig. 3E; p-value = 0.0053). The TCGA-TNBC cohort was subjected to Receiver Operating Characteristic (ROC) curve analysis, yielding an impressive area under the curve (AUC) of 0.949 (Fig. 3F). As expected, an increasing risk score was associated with unfavorable OS in TCGA-TNBC (Figs. 4A–4C) as well as METABRIC-TNBC cohort (Suppl. Figs. S6A–S6C).
Figure 4: Risk plot of immune least absolute shrinkage and selection operator (LASSO) Cox model for The Cancer Genome Atlas-Triple-Negative Breast Cancer (TCGA-TNBC). The upper dot plot displays increasing risk scores for low-risk (blue dots) or high risk (red dots) (A). The middle dot plot displays the OS data for cases ranked with the increasing risk score (B). The bottom heatmap summarizes the significant somatic mutations between low- and high-risk groups and the expression of 15 prioritized genes in the TCGA-TNBC (C).
Analyses of the differentially expressed genes (DEGs) between the LASSO triple-negative breast cancer risk clusters
In the TCGA-TNBC cohort, a DEG analysis was employed between the low and high-risk groups. In total, 681 DEGs were identified, meeting the criteria of Log2 fold change (Log2FC) ≥ 1 and a false discovery rate (FDR) ≤ 0.05. A total of 378 genes exhibited a higher expression in the low-risk cluster and 303 in the high-risk cluster (Suppl. Table S6). In 19 alphabetic × Cells molecular immune subtypes, significantly lower B-cells and plasma score were demonstrated in both high-risk subgroups of TCGA-TNBC as well as METABRIC-TNBC (Figs. 5A and 5B).
Figure 5: Transcription factor determination for high and low-risk group in The Cancer Genome Atlas-Triple-Negative Breast Cancer (TCGA-TNBC). (A) Box plot shows significantly lowered B-cell-score in the high-risk subgroup of TCGA-TNBC and METABRIC-TNBC. (B) The Box plot shows significant lower plasma cell-score in the high-risk subgroup of TCGA-TNBC and METABRIC-TNBC. (C) The network summarizes complex connections between transcription factors (green squares) and DEGs (red dots = up-regulated in the high-risk group; blue dots = up-regulated in low-risk group). (D) The copy number variation plot illustrates the relative frequency of copy number gains (in red) or deletions (in blue) within the high- and low-risk clusters of the TCGA-TNBC cohort. These differences are determined by Fisher’s exact test.
According to the results of the 681 DEGs, six significant UCSC_TFBSs (p ≤ 0.01) were identified: CHX10, FOXO3, POU6F1, S8, HNF1, and LHX3 using the DAVID algorithm (Fig. 5C).
Then, somatic mutation and CNV analyses were performed between the TNBC immune risk clusters. The top 300 high-frequency somatic mutations detected between the high- and low-risk clusters were analyzed. Four somatic mutations significantly differed between the two groups (Fig. 4C). They all demonstrated a higher frequency in the high-risk cluster (XIRP1, CACNA1C, USP9X, and CFAP46). In terms of CNV, several hot spots with a significant amplification in the copy number (chromosomes 2p, 2q, 7p, 7q, and 20q) or deletions (chromosomes 3p, 8p, 10q, 12q, 16q, 18p, 18q, and Xp) were observed as CNV characteristic features of the high-risk cluster in TCGA-TNBC cohort (Fig. 5D).
GSEA analysis considering the MSigDB, KEGG, and Hallmark datasets revealed that the top terms for the high-risk TCGA-TNBC cluster were KEGG_DRUG_METABOLISM_OTHER_ENZYMES and HALLMARK_EPITHELIAL_MESENCHYMAL_TRANSITION, whereas the top terms for the low-risk group were KEGG_ALLOGRAFT_REJECTION and HALLMARK_INTERFERON_GAMMA_RESPONSE (Figs. 6A and 6B; Suppl. Figs. S7A and S7B; Suppl. Tables S7 and S8).
Figure 6: Gene set enrichment analysis (GSEA) analyses for differentially expressed (DEGs) high and low risk The Cancer Genome Atlas-Triple-Negative Breast Cancer (TCGA-TNBC) clusters. GSEA of hallmark (A) and Kyoto Encyclopedia of Genes and Genomes (B) gene sets between high- and low-risk groups in TCGA-TNBC.
According to single-cell sequencing analysis, unfavorable significant Hallmark items, such as HALLMARK_EPITHELIAL_MESENCHYMAL_TRANSITION and HALLMARK_MYOGENESIS, were found to be prevalent in fibroblast cells and vascular smooth muscle cells (SMC). Conversely, favorable significant Hallmark items, including HALLMARK_E2F_TARGETS, HALLMARK_INFLAMMATORY_RESPONSE, HALLMARK_INTERFERON_ALPHA_RESPONSE, HALLMARK_ALLOGRAFT_REJECTION, and HALLMARK_INTERFERON_GAMMA_RESPONSE, were observed to be dominant in dendritic cell (DC), B cells, Tprolif, CD8Tex, and CD4Tconv (Fig. 7).
Figure 7: Single-cell sequencing analysis for significant Hallmark items in breast invasive carcinoma (BRCA; GSE176078). Uniform Manifold Approximation and Projection (UMAP) plots illustrate the activity of significant Hallmark items in indicated cell types from low (blue) to high (red).
Prediction of the sensitivities of chemotherapy and immunotherapy between the LASSO-TNBC risk clusters
Next, the prediction of either the resistance or the sensitivity of well-established chemotherapy and immunotherapy strategies in the high- and low risk-groups was examined. Based on the FDR, the top 30 chemotherapeutic agents between high- and low-risk clusters for the TCGA-TNBC (Fig. 8A; FDR < 0.01) and METABRIC-TNBC (Fig. 8B; FDR < 0.01) individually were selected. Eight chemotherapeutic compounds overlapped in both groups; seven of them (RO-3306, Tamoxifen, Sunitinib, JNK Inhibitor VIII, XMD11-85h, BX-912, and Tivozanib) were more sensitive in the high-risk group, and one (17-AAG) was more sensitive in the low-risk group. Six compounds enhanced sensitivity in the high-risk cluster with a significant positive correlation with the Rap1, Ras, and phosphoinositide 3-kinase/protein kinase B (PI3K-Akt) signaling pathways (Figs. 8C and 8D) based on the analysis of the STITCH database (XMD11-85h was not identified in the STITCH database).
Figure 8: Prediction of effective chemotherapy and immunotherapy for triple-negative breast cancer (TNBC) cohorts. Box plots comparing pRRophetic scores of the overlapped chemotherapy drugs between high- and low-risk groups in The Cancer Genome Atlas (TCGA)-TNBC (A) and Molecular Taxonomy of Breast Cancer International Consortium (METABRIC)-TNBC (B). A schematic representation of a protein-protein and protein-chemical association network is depicted for the high-risk sensitive overlapped chemotherapy, based on data extracted from the Search Tool for Interaction of Chemicals (STITCH) database (C). The enrichment pathways analysis of Kyoto Encyclopedia of Genes and Genomes for chemical associated proteins (D). Tumor Immune Dysfunction and Exclusion (TIDE) immunotherapy prediction analyses in TCGA-TNBC and METABRIC-TNBC (E and F).
Based on the TIDE prediction analysis of immunotherapy, the low-risk group demonstrated a higher immunotherapy responder rate than the high-risk group in both the TCGA-TNBC and METABRIC-TNBC cohorts (Figs. 8E and 8F). Four of the TIDE-predicted immunotherapy features were conspicuous in the high-risk cluster (Suppl. Figs. S8A–S8D: IFNG, Merck18, CD274, and CD8; all p-value < 0.05), and two were higher in the low-risk cluster (Suppl. Figs. S8E and S8F: Cancer-Associated Fibroblasts and Tumor-Associated Macrophage M2; all p-value < 0.05).
Employing the technique of single-cell sequencing analysis, the investigation unveiled the prevalence of three STITCH gene candidates (ESR1, ERBB2, and KIT) within malignant cells. Remarkably, five distinct STITCH gene candidates (FLT1, FLT3, FLT4, PDGFRA, and PDGFRB) exhibited a pronounced presence in endothelial cells, fibroblast cells, and Smooth Muscle Cells (SMC) (Fig. 9).
Figure 9: Single-cell sequencing analysis for significant Search Tool for Interaction of Chemicals (STITCH) gene candidates in breast invasive carcinoma (BRCA; GSE176078). Uniform Manifold Approximation and Projection (UMAP) plots illustrate the expression of STITCH gene candidates in indicated cell types from low (gray) to high (blue).
The Kras and PI3K-Akt signaling pathways exhibited higher activity levels in endothelial cells, fibroblast cells, SMC, dendritic cells, B cells, Tprolif, CD8Tex, and CD4Tconv (Fig. 10; Rap1 signaling is not available in TISCH2 online database).
Figure 10: Single-cell sequencing analysis for significant Search Tool for Interaction of Chemicals (STITCH) pathway items in breast invasive carcinoma (BRCA; GSE176078). Uniform Manifold Approximation and Projection (UMAP) plots illustrate the activity of significant STITCH pathway items in indicated cell types from low (blue) to high (red).
The IME of TNBC encapsulates a dynamic interplay between the subsets of immune cells and the molecular mediators, orchestrating a complex milieu that influences tumor progression and therapeutic responses (Zheng et al., 2021). This study unraveled 19 prominent xCells molecular immune subtypes in TNBC. Through WGCNA analysis, 410 TNBC immune candidate genes exhibiting strong positive correlations with the dominant xCells molecular immune subtypes in TCGA-TNBC and METABRIC-TNBC cohorts were identified. Furthermore, a novel immune prognostic stratification for TNBC based on a LASSO Cox regression model was devised, incorporating 15 genes. Notably, it was discovered that the high-risk TNBC immune groups exhibited increased sensitivity to various therapeutic agents. Furthermore, an analysis of the STITCH database revealed close associations between the high-risk cluster and key signaling pathways, such as Rap1, Ras, and PI3K-Akt. Finally, employing the TIDE immunotherapy prediction analysis, it was observed that the low-risk group displayed a higher immunotherapy response rate compared to the high-risk group in both the TCGA-TNBC and METABRIC-TNBC cohorts.
Based on the results of the xCells analysis, 19 TNBC prominent xCells molecular immune subtypes were identified, in which several subpopulations of B-cells were identified to be abundant. Numerous studies have consistently reported that TNBC tumors exhibit a higher infiltration of B-cells than other types of breast cancer. Owing to the recent approval of immunotherapeutic drugs as a treatment option in both early and metastatic settings for TNBC patients, the presence of B-cell populations or tertiary lymphoid structures holds promise as valuable biomarkers for predicting the immunotherapy responses within specific subgroups of breast cancer (Hu et al., 2021a; Li et al., 2023; Toney et al., 2022). Interestingly, we found higher TILs-B and plasma cells in high-risk cluster of TNBC. Many investigations on those B-cell subtypes infiltrating in TNBC are associated with a favorable prognosis in terms of relapse-free survival (RFS) and OS, increased sCD20+, sCD38+, and sCD138+ TILs were also linked to a favorable prognosis (Kuroda et al., 2021; Mohammed et al., 2013). Importantly, irrespective of breast cancer subtypes, the role of TILs-B and plasma cells is likely to be more complex than first considered, and further research is needed to shed light on their prognostic value in breast cancer. These findings highlight the potential importance of TILs-B in influencing the clinical outcome of TNBC (Lam and Verrill, 2023).
Our results show the lower immunotherapy responder rate in high-risk group with lower IFNG, Merck18, CD274, and CD8 levels, and higher cancer-associated fibroblasts and tumor-associated macrophage M2. On the other hand, an in silico drug screening system had identified six potentially effective compounds for the high-risk group. Tamoxifen, sunitinib, and tivozanib were significant chemical compounds in the STITCH network, which are medications used in the treatment of specific types of cancer. Tamoxifen, a selective ER modulator, is prescribed for hormone receptor-positive breast cancer patients to inhibit estrogen's effects and slow tumor growth (Davezac et al., 2023). Sunitinib, an oral tyrosine kinase inhibitor, targets multiple receptors involved in tumor growth and angiogenesis (Gundogdu et al., 2022). Similarly, Tivozanib, another oral tyrosine kinase inhibitor, specifically targets VEGFRs to hinder tumor blood vessel formation (Sakellakis and Zakopoulou, 2023). The STITCH database suggested that Rap1, Ras, and PI3K-Akt signaling pathways were crucial factors for establishing and maintaining the high-risk phenotype. Rap1, Ras, and the PI3K-Akt pathways are interconnected signaling cascades that regulate crucial cellular processes. Ras activates both Raf-MEK-ERK and PI3K-Akt pathways. Rap1, similar to Ras, can interact with it and activate the PI3K-Akt pathway. Additionally, these complex interactions play essential roles in cellular functions and have implications for disease treatments and drug development (de Luca et al., 2012; Zhang et al., 2022).
Mounting evidence substantiates the immune-modulatory impact of PI3K/AKT/mTOR and MAPK/MEK/ERK inhibitors, rendering them potentially attractive as adjuvants to immunotherapy for the treatment of the high-risk TNBC. Loi et al. (2016) demonstrated the activation of the Ras-MAPK pathway in TNBC was associated with reduced tumor-infiltrating lymphocytes and a poorer prognosis. The combined inhibition of MAPK and PD-L1/PD-1 demonstrated high potential for enhancing the anti-tumor immune responses in TNBC, offering a promising therapeutic strategy (Loi et al., 2016). Preclinical studies have shown that inhibition of the MAPK pathway can effectively reverse various immunosuppressive processes, including increased infiltration of regulatory T cells (Tregs), accumulation of myeloid-derived suppressor cells (MDSCs), impaired tumor antigen presentation, reduced release of key effector cytokines such as IFN, IL-2, and TNF, as well as elevated expression of immunosuppressive factors like IL-10 and CCL2 (Ho et al., 2014). Similar to the MAPK pathway, In the realm of immunosuppression, PI3K signaling plays a pivotal role, with PTEN loss contributing to an upsurge in PD-L1 expression. This, in turn, fosters the recruitment of immune regulatory elements like MDSCs and Tregs (Zhang and Richmond, 2021). In a clinical trial, a PI3K/mTOR dual inhibitor was combined with immune checkpoint inhibitors demonstrated synergy, enhancing growth inhibition and increased activation of T-cell, natural killer-cell, and dendritic cell-responses (Yan et al., 2021).
Like other studies that integrate sequencing data from bulk tumor tissue, our research has several inherent limitations. For instance, a prospective analysis of a tremendously large cohort of patients with TNBC is needed to validate the LASSO Cox risk model. Furthermore, the effectiveness of targeting the Rap1, Ras, and PI3K-Akt signaling pathways for treating high risk TNBC requires further validation in appropriate preclinical models and future clinical trials. These findings emphasize the need for continued investigation to fully elucidate the potential clinical implications of these pathways in TNBC.
In summary, 410 hub TNBC immune-related candidate genes were identified by WGCNA, and 34 potential prognostic candidates were identified by Univariate Cox regression in the TCGA-TNBC cohort. Then, 15 candidates were prioritized by the LASSO Cox risk regression model, and these genes were employed to identify low and high-risk subgroups and unfavorable survival. In silico drug screening identified six effective compounds for high-risk TNBC. Furthermore, the STITCH database highlighted Rap1, Ras, and PI3K-Akt signaling as potential targets for controlling high-risk TNBC. This study suggests a molecular immune classifier for TNBC, enabling the development of novel diagnostic approaches and precision treatments that exploit the immunological intricacies of this challenging disease. Future aspects encompass experimental validation, clinical studies, mechanistic insights, drug development, personalized medicine, immunotherapy exploration, and multi-omics integration, all of which could significantly advance TNBC diagnostics and treatment strategies.
Acknowledgement: We extend our heartfelt appreciation to the participants of the TCGA-BRCA and METABRIC-BRCA cohorts for providing their clinical information and mRNA expression data, which were crucial for our analysis. We also thank the researchers and staff involved in data collection and curation.
Funding Statement: The authors received no specific funding for this study.
Author Contributions: Study conception and design: Kuailu Lin, Xixi Lai; Data collection: Kuailu Lin, Qianyu Gu; Analysis and interpretation of results: Kuailu Lin, Qianyu Gu, Xixi Lai; Draft manuscript preparation: Kuailu Lin, Xixi Lai.
Availability of Data and Materials: The datasets generated during and/or analysed during the current study are available from the corresponding author on reasonable request.
Ethics Approval: METABRIC and TCGA belong to public databases. The patients involved in the database have obtained ethical approval. Users can download relevant data for free for research and publish relevant articles. Our study is based on open-access data, so there are no ethical issues and other conflicts of interest.
Conflicts of Interest: The authors declare that they have no conflicts of interest to report regarding the present study.
Supplementary Materials: The supplementary material is available online at https://doi.org/10.32604/biocell.2023.043298.
References
Almansour NM (2022). Triple-negative breast cancer: A brief review about epidemiology, risk factors, signaling pathways, treatment and role of artificial intelligence. Frontiers in Molecular Biosciences 9: 836417. https://doi.org/10.3389/fmolb.2022.836417 [Google Scholar] [PubMed] [CrossRef]
Aran D, Hu Z, Butte AJ (2017). xCell: Digitally portraying the tissue cellular heterogeneity landscape. Genome Biology 18: 220. https://doi.org/10.1186/s13059-017-1349-1 [Google Scholar] [PubMed] [CrossRef]
Burstein MD, Tsimelzon A, Poage GM, Covington KR, Contreras A et al. (2015). Comprehensive genomic analysis identifies novel subtypes and targets of triple-negative breast cancer. Clinical Cancer Research 21: 1688–1698. https://doi.org/10.1158/1078-0432.CCR-14-0432 [Google Scholar] [PubMed] [CrossRef]
Davezac M, Meneur C, Buscato M, Zahreddine R, Arnal JF, Dalenc F, Lenfant F, Fontaine C (2023). The beneficial effects of tamoxifen on arteries: A key player for cardiovascular health of breast cancer patient. Biochemical Pharmacology 214: 115677. https://doi.org/10.1016/j.bcp.2023.115677 [Google Scholar] [PubMed] [CrossRef]
de Luca A, Maiello MR, D’Alessio A, Pergameno M, Normanno N (2012). The RAS/RAF/MEK/ERK and the PI3K/AKT signalling pathways: Role in cancer pathogenesis and implications for therapeutic approaches. Expert Opinion on Therapeutic Targets 16: S17–27. https://doi.org/10.1517/14728222.2011.639361 [Google Scholar] [PubMed] [CrossRef]
Derakhshan F, Reis-Filho JS (2022). Pathogenesis of triple-negative breast cancer. Annual Review of Pathology-Mechanisms of Disease 17: 181–204. https://doi.org/10.1146/annurev-pathol-042420-093238 [Google Scholar] [PubMed] [CrossRef]
Dietze EC, Sistrunk C, Miranda-Carboni G, O’Regan R, Seewaldt VL (2015). Triple-negative breast cancer in African-American women: Disparities versus biology. Nature Reviews Cancer 15: 248–254. https://doi.org/10.1038/nrc3896 [Google Scholar] [PubMed] [CrossRef]
Ding S, Qiao N, Zhu Q, Tong Y, Wang S, Chen X, Tian Q, Xiao Y, Shen K (2023). Single-cell atlas reveals a distinct immune profile fostered by T cell-B cell crosstalk in triple negative breast cancer. Cancer Communications 43: 661–684. https://doi.org/10.1002/cac2.12429 [Google Scholar] [PubMed] [CrossRef]
Dore-Savard L, Lee E, Kakkad S, Popel AS, Bhujwalla ZM (2016). The angiogenic secretome in VEGF overexpressing breast cancer xenografts. Scientific Reports 6: 39460. https://doi.org/10.1038/srep39460 [Google Scholar] [PubMed] [CrossRef]
Ensenyat-Mendez M, Llinas-Arias P, Orozco JIJ, Iniguez-Munoz S, Salomon MP, Sese B, DiNome ML, Marzese DM (2021). Current triple-negative breast cancer subtypes: Dissecting the most aggressive form of breast cancer. Frontiers in Oncology 11: 681476. https://doi.org/10.3389/fonc.2021.681476 [Google Scholar] [PubMed] [CrossRef]
Fan Y, He S (2022). The characteristics of tumor microenvironment in triple negative breast cancer. Cancer Management and Research 14: 1–17. https://doi.org/10.2147/CMAR.S316700 [Google Scholar] [PubMed] [CrossRef]
Geeleher P, Cox N, Huang RS (2014). pRRophetic: An R package for prediction of clinical chemotherapeutic response from tumor gene expression levels. PLoS One 9: e107468. https://doi.org/10.1371/journal.pone.0107468 [Google Scholar] [PubMed] [CrossRef]
Grivennikov SI, Greten FR, Karin M (2010). Immunity, inflammation, and cancer. Cell 140: 883–899. https://doi.org/10.1016/j.cell.2010.01.025 [Google Scholar] [PubMed] [CrossRef]
Gundogdu Y, Deniz OC, Saka D, Sisman G, Erdamar S, Koksal I (2022). Sunitinib induced colitis manifesting as invasive diarrhea in a patient with renal cell carcinoma. Journal of Oncology Pharmacy Practice 28: 516–518. https://doi.org/10.1177/10781552211061183 [Google Scholar] [PubMed] [CrossRef]
Han Y, Wang Y, Dong X, Sun D, Liu Z, Yue J, Wang H, Li T, Wang C (2023). TISCH2: Expanded datasets and new tools for single-cell transcriptome analyses of the tumor microenvironment. Nucleic Acids Research 51: D1425–D1431. https://doi.org/10.1093/nar/gkac959 [Google Scholar] [PubMed] [CrossRef]
Ho PC, Meeth KM, Tsui YC, Srivastava B, Bosenberg MW, Kaech SM (2014). Immune-based antitumor effects of BRAF inhibitors rely on signaling by CD40L and IFNgamma. Cancer Research 74: 3205–3217. https://doi.org/10.1158/0008-5472.CAN-13-3461 [Google Scholar] [PubMed] [CrossRef]
Huang DW, Sherman BT, Lempicki RA (2009). Systematic and integrative analysis of large gene lists using DAVID bioinformatics resources. Nature Protocols 4: 44–57. https://doi.org/10.1038/nprot.2008.211 [Google Scholar] [PubMed] [CrossRef]
Hu Q, Hong Y, Qi P, Lu G, Mai X et al. (2021a). Atlas of breast cancer infiltrated B-lymphocytes revealed by paired single-cell RNA-sequencing and antigen receptor profiling. Nature Communications 12: 2186. https://doi.org/10.1038/s41467-021-22300-2 [Google Scholar] [PubMed] [CrossRef]
Hu S, Qu X, Jiao Y, Hu J, Wang B (2021b). Immune Classification and immune landscape analysis of triple-negative breast cancer. Frontiers in Genetics 12: 710534. https://doi.org/10.3389/fgene.2021.710534 [Google Scholar] [PubMed] [CrossRef]
Hudis CA, Gianni L (2011). Triple-negative breast cancer: An unmet medical need. Oncologist 16: 1–11. https://doi.org/10.1634/theoncologist.2011-S1-01 [Google Scholar] [PubMed] [CrossRef]
Kuroda H, Jamiyan T, Yamaguchi R, Kakumoto A, Abe A, Harada O, Enkhbat B, Masunaga A (2021). Prognostic value of tumor-infiltrating B lymphocytes and plasma cells in triple-negative breast cancer. Breast Cancer 28: 904–914. https://doi.org/10.1007/s12282-021-01227-y [Google Scholar] [PubMed] [CrossRef]
Lam BM, Verrill C (2023). Clinical significance of tumour-infiltrating B lymphocytes (TIL-Bs) in Breast cancer: A systematic literature review. Cancers 15: 1164. https://doi.org/10.3390/cancers15041164 [Google Scholar] [PubMed] [CrossRef]
Langfelder P, Horvath S (2008). WGCNA: An R package for weighted correlation network analysis. BMC Bioinformatics 9: 559. https://doi.org/10.1186/1471-2105-9-559 [Google Scholar] [PubMed] [CrossRef]
Larsen SJ, do Canto LM, Rogatto SR, Baumbach J (2018). CoNVaQ: A web tool for copy number variation-based association studies. BMC Genomics 19: 369. https://doi.org/10.1186/s12864-018-4732-8 [Google Scholar] [PubMed] [CrossRef]
Lee E, Fertig EJ, Jin K, Sukumar S, Pandey NB, Popel AS (2014). Breast cancer cells condition lymphatic endothelial cells within pre-metastatic niches to promote metastasis. Nature Communications 5: 4715. https://doi.org/10.1038/ncomms5715 [Google Scholar] [PubMed] [CrossRef]
Li Z, Qiu Y, Lu W, Jiang Y, Wang J (2018). Immunotherapeutic interventions of triple negative breast cancer. Journal of Translational Medicine 16: 147. https://doi.org/10.1186/s12967-018-1514-7 [Google Scholar] [PubMed] [CrossRef]
Li M, Quintana A, Alberts E, Hung MS, Boulat V, Ripoll MM, Grigoriadis A (2023). B Cells in breast cancer pathology. Cancers 15: 1517. https://doi.org/10.3390/cancers15051517 [Google Scholar] [PubMed] [CrossRef]
Liu F, Lang R, Zhao J, Zhang X, Pringle GA, Fan Y, Yin D, Gu F, Yao Z, Fu L (2011). CD8+ cytotoxic T cell and FOXP3+ regulatory T cell infiltration in relation to breast cancer survival and molecular subtypes. Breast Cancer Research and Treatment 130: 645–655. https://doi.org/10.1007/s10549-011-1647-3 [Google Scholar] [PubMed] [CrossRef]
Loi S, Dushyanthen S, Beavis PA, Salgado R, Denkert C et al. (2016). RAS/MAPK activation is associated with reduced tumor-infiltrating lymphocytes in triple-negative breast cancer: Therapeutic cooperation between MEK and PD-1/PD-L1 immune checkpoint inhibitors. Clinical Cancer Research 22: 1499–1509. https://doi.org/10.1158/1078-0432.CCR-15-1125 [Google Scholar] [PubMed] [CrossRef]
McCarthy DJ, Chen Y, Smyth GK (2012). Differential expression analysis of multifactor RNA-Seq experiments with respect to biological variation. Nucleic Acids Research 40: 4288–4297. https://doi.org/10.1093/nar/gks042 [Google Scholar] [PubMed] [CrossRef]
Miyashita M, Sasano H, Tamaki K, Hirakawa H, Takahashi Y et al. (2015). Prognostic significance of tumor-infiltrating CD8+ and FOXP3+ lymphocytes in residual tumors and alterations in these parameters after neoadjuvant chemotherapy in triple-negative breast cancer: A retrospective multicenter study. Breast Cancer Research 17: 124. https://doi.org/10.1186/s13058-015-0632-x [Google Scholar] [PubMed] [CrossRef]
Mohammed ZM, Going JJ, Edwards J, Elsberger B, McMillan DC (2013). The relationship between lymphocyte subsets and clinico-pathological determinants of survival in patients with primary operable invasive ductal breast cancer. British Journal of Cancer 109: 1676–1684. https://doi.org/10.1038/bjc.2013.493 [Google Scholar] [PubMed] [CrossRef]
Nolan E, Lindeman GJ, Visvader JE (2023). Deciphering breast cancer: From biology to the clinic. Cell 186: 1708–1728. https://doi.org/10.1016/j.cell.2023.01.040 [Google Scholar] [PubMed] [CrossRef]
Pan K, Guan XX, Li YQ, Zhao JJ, Li JJ et al. (2014). Clinical activity of adjuvant cytokine-induced killer cell immunotherapy in patients with post-mastectomy triple-negative breast cancer. Clinical Cancer Research 20: 3003–3011. https://doi.org/10.1158/1078-0432.CCR-14-0082 [Google Scholar] [PubMed] [CrossRef]
Park JH, Ahn JH, Kim SB (2018). How shall we treat early triple-negative breast cancer (TNBCFrom the current standard to upcoming immuno-molecular strategies. ESMO Open 3: e000357. https://doi.org/10.1136/esmoopen-2018-000357 [Google Scholar] [PubMed] [CrossRef]
Robinson JT, Thorvaldsdottir H, Wenger AM, Zehir A, Mesirov JP (2017). Variant review with the integrative genomics viewer. Cancer Research 77: e31–e34. https://doi.org/10.1158/0008-5472.CAN-17-0337 [Google Scholar] [PubMed] [CrossRef]
Romero-Cordoba S, Meneghini E, Sant M, Iorio MV, Sfondrini L, Paolini B, Agresti R, Tagliabue E, Bianchi F (2019). Decoding immune heterogeneity of triple negative breast cancer and its association with systemic inflammation. Cancers 11: 911. https://doi.org/10.3390/cancers11070911 [Google Scholar] [PubMed] [CrossRef]
Sakellakis M, Zakopoulou R (2023). Current status of tivozanib in the treatment of patients with advanced renal cell carcinoma. Cureus 15: e35675. https://doi.org/10.7759/cureus.35675 [Google Scholar] [PubMed] [CrossRef]
Santoni M, Romagnoli E, Saladino T, Foghini L, Guarino S, Capponi M, Giannini M, Cognigni PD, Ferrara G, Battelli N (2018). Triple negative breast cancer: Key role of tumor-associated macrophages in regulating the activity of anti-PD-1/PD-L1 agents. Biochimica et Biophysica Acta (BBA)-Reviews on Cancer 1869: 78–84. https://doi.org/10.1016/j.bbcan.2017.10.007 [Google Scholar] [PubMed] [CrossRef]
Shannon P, Markiel A, Ozier O, Baliga NS, Wang JT, Ramage D, Amin N, Schwikowski B, Ideker T (2003). Cytoscape: A software environment for integrated models of biomolecular interaction networks. Genome Research 13: 2498–2504. https://doi.org/10.1101/gr.1239303 [Google Scholar] [PubMed] [CrossRef]
Sherman BT, Hao M, Qiu J, Jiao X, Baseler MW, Lane HC, Imamichi T, Chang W (2022). DAVID: A web server for functional enrichment analysis and functional annotation of gene lists (2021 update). Nucleic Acids Research 50: W216–W221. https://doi.org/10.1093/nar/gkac194 [Google Scholar] [PubMed] [CrossRef]
Siegel RL, Miller KD, Jemal A (2018). Cancer statistics, 2018. CA: A Cancer Journal for Clinicians 68: 7–30. https://doi.org/10.3322/caac.21442 [Google Scholar] [PubMed] [CrossRef]
Solinas C, Gombos A, Latifyan S, Piccart-Gebhart M, Kok M, Buisseret L (2017). Targeting immune checkpoints in breast cancer: An update of early results. ESMO Open 2: e000255. https://doi.org/10.1136/esmoopen-2017-000255 [Google Scholar] [PubMed] [CrossRef]
Szklarczyk D, Gable AL, Lyon D, Junge A, Wyder S et al. (2019). STRING v11: Protein-protein association networks with increased coverage, supporting functional discovery in genome-wide experimental datasets. Nucleic Acids Research 47: D607–D613. https://doi.org/10.1093/nar/gky1131 [Google Scholar] [PubMed] [CrossRef]
Testa U, Castelli G, Pelosi E (2020). Breast cancer: A molecularly heterogenous disease needing subtype-specific treatments. Medical Sciences 8: 18. https://doi.org/10.3390/medsci8010018 [Google Scholar] [PubMed] [CrossRef]
Tibshirani R (1997). The lasso method for variable selection in the Cox model. Statistics in Medicine 16: 385–395. https://doi.org/10.1002/(ISSN)1097-0258 [Google Scholar] [CrossRef]
Toney NJ, Opdenaker LM, Cicek K, Frerichs L, Kennington CR, Oberly S, Archinal H, Somasundaram R, Sims-Mourtada J (2022). Tumor-B-cell interactions promote isotype switching to an immunosuppressive IgG4 antibody response through upregulation of IL-10 in triple negative breast cancers. Journal of Translational Medicine 20: 112. https://doi.org/10.1186/s12967-022-03319-5 [Google Scholar] [PubMed] [CrossRef]
Tsang JYS, Tse GM (2020). Molecular classification of breast cancer. Advances in Anatomic Pathology 27: 27–35. https://doi.org/10.1097/PAP.0000000000000232 [Google Scholar] [PubMed] [CrossRef]
van den Ende NS, Nguyen AH, Jager A, Kok M, Debets R, van Deurzen CHM (2023). Triple-negative breast cancer and predictive markers of response to neoadjuvant chemotherapy: A systematic review. International Journal of Molecular Sciences 24: 2969. https://doi.org/10.3390/ijms24032969 [Google Scholar] [PubMed] [CrossRef]
Vikas P, Borcherding N, Zhang W (2018). The clinical promise of immunotherapy in triple-negative breast cancer. Cancer Management and Research 10: 6823–6833. https://doi.org/10.2147/CMAR [Google Scholar] [CrossRef]
Wilkerson MD, Hayes DN (2010). ConsensusClusterPlus: A class discovery tool with confidence assessments and item tracking. Bioinformatics 26: 1572–1573. https://doi.org/10.1093/bioinformatics/btq170 [Google Scholar] [PubMed] [CrossRef]
Wu SZ, Al-Eryani G, Roden DL, Junankar S, Harvey K et al. (2021a). A single-cell and spatially resolved atlas of human breast cancers. Nature Genetics 53: 1334–1347. https://doi.org/10.1038/s41588-021-00911-1 [Google Scholar] [PubMed] [CrossRef]
Wu T, Hu E, Xu S, Chen M, Guo P et al. (2021b). clusterProfiler 4.0: A universal enrichment tool for interpreting omics data. The Innovation 2: 100141. https://doi.org/10.1016/j.xinn.2021.100141 [Google Scholar] [PubMed] [CrossRef]
Yan C, Yang J, Saleh N, Chen SC, Ayers GD, Abramson VG, Mayer IA, Richmond A (2021). Inhibition of the PI3K/mTOR pathway in breast cancer to enhance response to immune checkpoint inhibitors in breast cancer. International Journal of Molecular Sciences 22: 5207. https://doi.org/10.3390/ijms22105207 [Google Scholar] [PubMed] [CrossRef]
Yoshihara K, Shahmoradgoli M, Martinez E, Vegesna R, Kim H et al. (2013). Inferring tumour purity and stromal and immune cell admixture from expression data. Nature Communications 4: 2612. https://doi.org/10.1038/ncomms3612 [Google Scholar] [PubMed] [CrossRef]
Zhang Z, Richmond A (2021). The role of PI3K inhibition in the treatment of breast cancer, alone or combined with immune checkpoint inhibitors. Frontiers in Molecular Biosciences 8: 648663. https://doi.org/10.3389/fmolb.2021.648663 [Google Scholar] [PubMed] [CrossRef]
Zhang Z, Richmond A, Yan C (2022). Immunomodulatory properties of PI3K/AKT/mTOR and MAPK/MEK/ERK inhibition augment response to immune checkpoint blockade in melanoma and triple-negative breast cancer. International Journal of Molecular Sciences 23: 7353. https://doi.org/10.3390/ijms23137353 [Google Scholar] [PubMed] [CrossRef]
Zheng H, Siddharth S, Parida S, Wu X, Sharma D (2021). Tumor microenvironment: Key players in triple negative breast cancer immunomodulation. Cancers 13: 3357. https://doi.org/10.3390/cancers13133357 [Google Scholar] [PubMed] [CrossRef]
Cite This Article
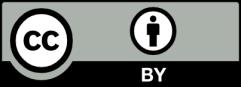