Open Access
ARTICLE
A Novel Krill Herd Based Random Forest Algorithm for Monitoring Patient Health
1 Department of Health Information Management and Technology, University of Hafr Al-Batin, Saudi Arabia
2 Department of Electrical and Computer Engineering, Faculty of Engineering, King Abdulaziz, Jeddah, 21589, Saudi Arabia
3 Center of Excellence in Intelligent Engineering Systems, King Abdul Aziz University, Jeddah, Saudi Arabia
4 College of Medicine, King Khalid University, Abha, Saudi Arabia
5 Department of Physics, College of Science, Jazan University, P.O. Box. 114, Jazan, 45142, Saudi Arabia
6 Department of Biology, College of Science, Imam Mohammad Ibn Saud Islamic University (IMSIU), Riyadh, 11623, Saudi Arabia
7 Computer Science Department, Faculty of Computing and Information Technology, King Abdulaziz University, Jeddah, 21589, Saudi Arabia
8 Department of Math and Computer Science, Augustana College, IL, 61201, United States
* Corresponding Author: Tauheed Khan Mohd. Email:
Computers, Materials & Continua 2023, 75(2), 4553-4571. https://doi.org/10.32604/cmc.2023.032118
Received 07 May 2022; Accepted 19 November 2022; Issue published 31 March 2023
Abstract
Artificial Intelligence (AI) is finding increasing application in healthcare monitoring. Machine learning systems are utilized for monitoring patient health through the use of IoT sensor, which keep track of the physiological state by way of various health data. Thus, early detection of any disease or derangement can aid doctors in saving patients’ lives. However, there are some challenges associated with predicting health status using the common algorithms, such as time requirements, chances of errors, and improper classification. We propose an Artificial Krill Herd based on the Random Forest (AKHRF) technique for monitoring patients’ health and eliciting an optimal prescription based on their health status. To begin with, various patient datasets were collected and trained into the system using IoT sensors. As a result, the framework developed includes four processes: preprocessing, feature extraction, classification, and result visibility. Additionally, preprocessing removes errors, noise, and missing values from the dataset, whereas feature extraction extracts the relevant information. Then, in the classification layer, we updated the fitness function of the krill herd to classify the patient’s health status and also generate a prescription. We found that the results from the proposed framework are comparable to the results from other state-of-the-art techniques in terms of sensitivity, specificity, Area under the Curve (AUC), accuracy, precision, recall, and F-measure.Keywords
Cite This Article
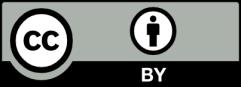