Open Access
ABSTRACT
Convolution Neural Networks and Support Vector Machines for Automatic Segmentation of Intracoronary Optical Coherence Tomography
1 School of Biological Science & Medical Engineering, Southeast University, Nanjing 210096, China.
2 School of Information Science & Engineering, Yunnan University, Kunming 650091, China.
3 School of Mathematics, Southeast University, Nanjing 210096, China.
4 Department of Medicine, Emory University School of Medicine, Atlanta, GA 30307, USA.
5 The Wallace H. Coulter Department of Biomedical Engineering, Georgia Institute of Technology, Atlanta, GA 30332, USA.
6 Institute for Medical Engineering & Science, Massachusetts Institute of Technology, Cambridge, MA 02139, USA
7 Mathematical Sciences Department, Worcester Polytechnic Institute, Worcester, MA 01609, USA.
* Corresponding authors: Dalin Tang, dtang@wpi.edu; Rencan Nei, rcnie@ynu.edu.cn; Jinde Cao, jdcao@seu.edu.cn.
Molecular & Cellular Biomechanics 2019, 16(Suppl.2), 31-31. https://doi.org/10.32604/mcb.2019.06983
Abstract
Cardiovascular diseases are closely associated with deteriorating atherosclerotic plaques. Optical coherence tomography (OCT) is a recently developed intravascular imaging technique with high resolution approximately 10 microns and could provide accurate quantification of coronary plaque morphology. However, tissue segmentation of OCT images in clinic is still mainly performed manually by physicians which is time consuming and subjective. To overcome these limitations, two automatic segmentation methods for intracoronary OCT image based on support vector machine (SVM) and convolutional neural network (CNN) were performed to identify the plaque region and characterize plaque components. In vivo IVUS and OCT coronary plaque data from 5 patients were acquired at Emory University with patient’s consent obtained. Seventy-seven matched IVUS and OCT slices with good image quality and lipid cores were selected for this study. Manual OCT segmentation was performed by experts using virtual histology IVUS guidance, and used as gold standard in the automatic segmentations. The overall classification accuracy based on CNN method reached 95.8%, and the accuracy based on SVM was 71.9%. The CNN-based segmentation method can better characterize plaque compositions on OCT images and greatly reduce the time spent by doctors in segmenting and identifying plaques.Keywords
Cite This Article
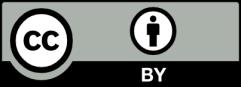